The world is rapidly moving towards renewable energy sources instead of conventional ones, but here’s the first stumbling point—the integration of renewable energy sources into traditional energy management systems.
The challenge lies in effectively managing the intermittent nature of renewable energy sources such as solar and wind power. Traditional energy management systems are designed to handle consistent and predictable energy generation and consumption patterns.
However, renewable energy sources fluctuate based on weather conditions and other factors, making it crucial to develop AI-powered solutions that can optimize the integration of these sources into the existing energy grid.
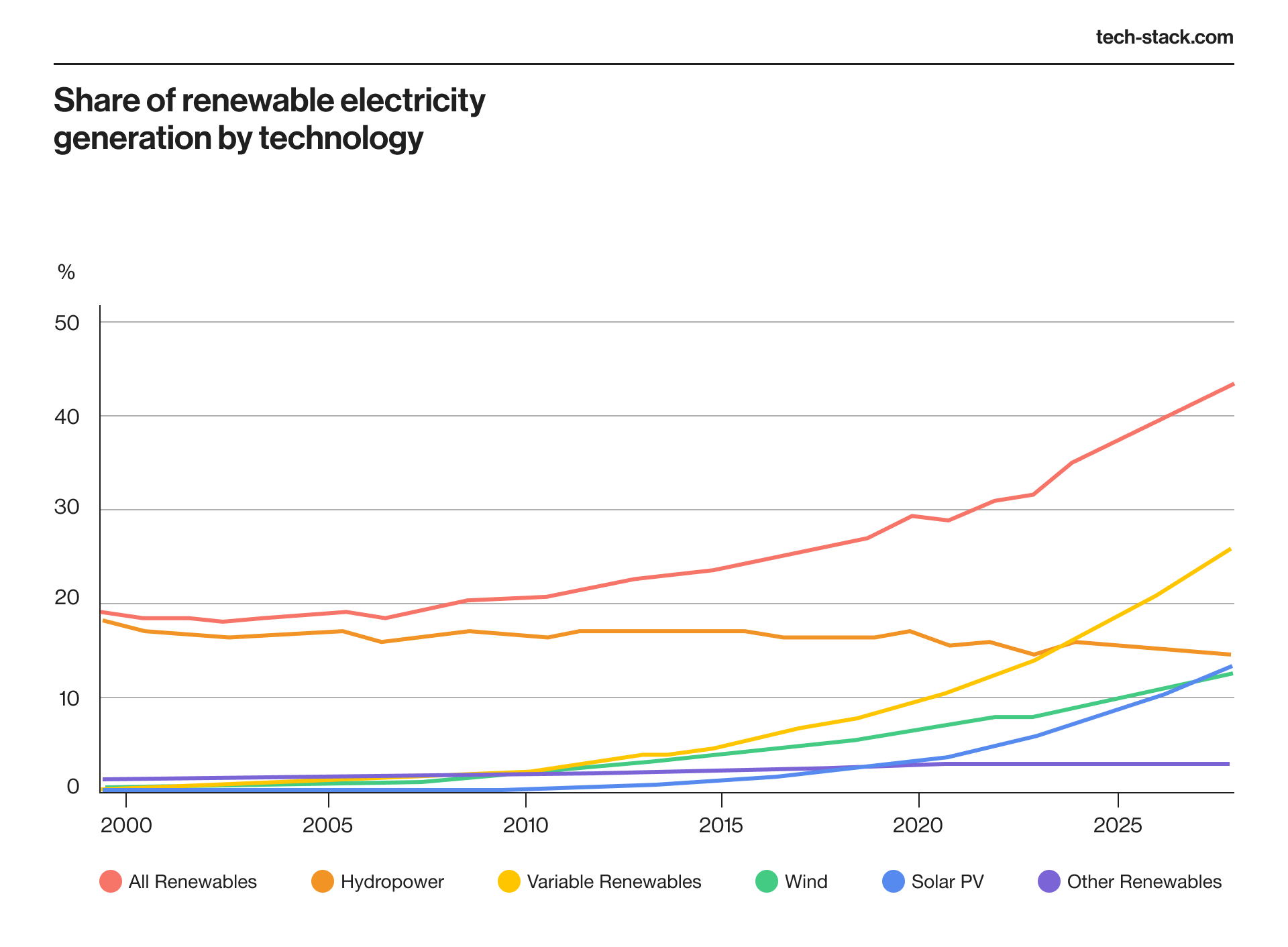
In 2022, non-bioenergy renewable sources contributed to nearly 30% of electricity generation globally. This significant shift towards renewable energy highlights the growing importance of integrating these sources into traditional energy management systems. By 2028, the global power mix will be transformed, taking into account the recent progress.
The deployment of AI in smart grid management enables real-time monitoring and decision-making, optimizing the balance between energy supply and demand. This is achieved through sophisticated forecasting models that predict energy production from renewable sources and adjust the grid's operations accordingly. Such predictive capabilities are essential for maintaining grid stability, especially during peak demand times or when renewable energy generation drops unexpectedly.
AI-powered smart grid management not only enhances grid stability and efficiency, but also supports the transition towards a more sustainable energy system. It achieves this by maximizing the utilization of renewable energy sources, reducing reliance on fossil fuels, and consequently decreasing carbon emissions to mitigate the impact of climate change.
As a software engineer with hands-on experience with renewable energy, I’d like to share my insights on how AI can improve grid management.
AI's Role in Renewable Energy and Grid Management
AI plays a pivotal role in transforming renewable energy and grid management, offering innovative solutions to some of the most pressing challenges in the sector. Its impact spans several key areas:
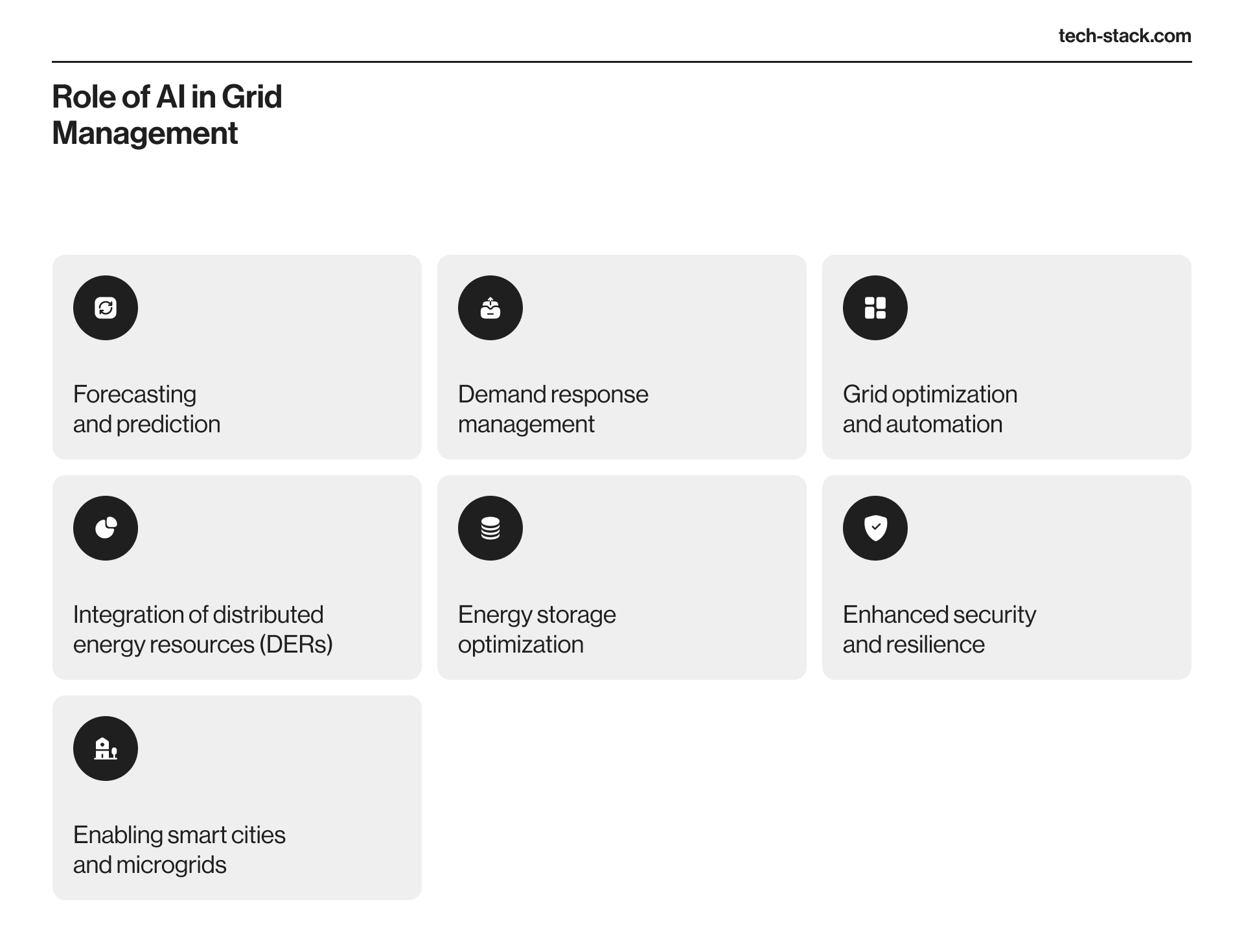
Forecasting and prediction
AI significantly improves the accuracy of forecasting renewable energy production, such as solar and wind power. By analyzing vast amounts of historical weather data and real-time information, AI algorithms can predict energy output with high precision.
Sure, AI models are effective in forecasting and can be further enhanced by integrating them with tools for solving differential equations. However, when it comes to creating a model that predicts energy levels with high precision, we usually utilize APIs from established forecasting models to obtain weather data. This weather data serves as a crucial input for our predictive model, enabling more accurate and reliable energy forecasts.
Such an approach combines the strengths of advanced AI algorithms and real-time environmental data, thereby optimizing the model's predictive capabilities. This capability is crucial for grid operators to plan energy distribution and ensure reliability, especially given the variable nature of renewable energy sources.
Demand response management
Demand response programs are essential for maintaining grid stability by adjusting consumer energy consumption during peak hours. AI enables more sophisticated and automated demand response strategies by predicting peak load periods and optimizing energy distribution accordingly. It can also engage consumers through smart home devices, adjusting energy usage in real-time based on grid conditions.
Grid optimization and automation
AI enhances grid optimization by continuously analyzing data from smart meters, sensors, and IoT devices across the grid. With custom AI development services, your software system will be able to identify patterns, predict potential failures or outages, and autonomously reconfigure the grid to prevent disruptions. This level of automation not only improves efficiency, but also extends the lifespan of grid infrastructure.
Integration of distributed energy resources (DERs)
DERs, including rooftop solar panels, wind turbines, and battery storage, are increasingly common. AI facilitates the integration of these resources into the grid by managing their variable output and ensuring that energy produced locally can be used effectively. This reduces transmission losses and leverages renewable energy more efficiently.
Energy storage optimization
Energy storage systems are vital for mitigating the intermittent nature of renewable energy. AI algorithms optimize the charging and discharging cycles of these storage systems, ensuring energy is available when production is low or demand is high. This optimization helps maximize the utility of stored energy, reduce costs, and increase the penetration of renewable sources in the energy mix.
Enhanced security and resilience
AI contributes to the security and resilience of the energy grid by monitoring for cyber threats and physical failures. Machine learning models can detect anomalies that indicate potential security breaches or system faults, enabling preemptive action to mitigate risks and ensure continuous energy supply.
Enabling smart cities and microgrids
AI is a key enabler of smart cities and microgrids, which are small-scale power grids that can operate independently or in conjunction with the main grid. AI manages and optimizes energy production, distribution, and consumption within these systems, promoting efficiency and sustainability.
AI's role in renewable energy and grid management is transformative, addressing the challenges of integrating renewable sources into the energy mix while enhancing grid stability, efficiency, and resilience. As AI technologies continue to evolve, their application in the energy sector promises to accelerate the transition to a sustainable, reliable, and intelligent energy system. The ongoing innovation in AI-driven solutions underscores the potential for a future where renewable energy can fully meet global energy needs.
The integration of AI in the energy sector is not only a leap towards modernization, but also a necessary evolution to address the increasing complexities and demands of global energy management. Software engineers working within the renewable energy sector know how to revolutionize this sector by offering solutions that can significantly enhance operational efficiency, sustainability, and resilience. However, realizing this potential requires overcoming a set of multifaceted challenges, ranging from technical hurdles to regulatory landscapes. Here is an expanded view of how AI can navigate these obstacles:
Expanding on Challenges and AI Solutions
Data management and quality
Challenge: The sheer volume of data from various sources like smart meters, sensors, and satellite imagery can be overwhelming. Inconsistencies, inaccuracies, and lack of standardization compound the issue, making data less reliable for critical decision-making.
AI solution: Advanced AI algorithms and machine learning techniques can automate the process of cleaning and standardizing data, ensuring high-quality datasets. These algorithms can also uncover insights from unstructured data, unlocking new opportunities for energy optimization.
Integration with legacy systems
Challenge: The energy sector's reliance on older, inflexible infrastructure can severely limit the integration and scalability of AI solutions, posing a significant barrier to innovation.
AI solution: AI can offer middleware solutions that act as a bridge between legacy systems and new technologies. These solutions can translate data formats and protocols, allowing for seamless communication and integration without the need for extensive infrastructure overhauls.
Cybersecurity threats
Challenge: The increasing digitization and interconnectivity of energy systems elevate the risk of cyberattacks, potentially jeopardizing the security of critical infrastructure.
AI solution: AI-enhanced cybersecurity measures can provide real-time monitoring and threat detection, using predictive analytics to identify potential vulnerabilities and threats before they manifest. AI can also automate responses to security incidents, reducing the time and resources required to address cyberattacks.
Regulatory and privacy concerns
Challenge: Compliance with evolving regulations and standards for data privacy and energy management can be daunting, especially when deploying new technologies like AI.
AI solution: AI systems can be designed with compliance in mind, incorporating regulatory requirements into their algorithms. Automated compliance tools powered by AI can help energy companies navigate complex legal landscapes, ensuring that operations remain within regulatory bounds.
Skill gaps and workforce transition
Challenge: The transition towards AI-driven energy systems necessitates a workforce skilled in both AI and energy management, a combination that is currently scarce.
AI solution: Comprehensive education and training programs, developed in partnership with academic institutions and technology companies, can equip current and future employees with the necessary skills. AI can also assist in this educational endeavor, providing personalized learning experiences and simulations.
High initial costs
Challenge: The investment required for AI implementation, including the technology itself and the infrastructure to support it, can be significant, deterring smaller operators from adoption.
AI solution: Cloud-based AI services and as-a-service models can lower the barrier to entry, offering scalable and cost-effective solutions. These models allow companies to pay for AI capabilities as they use them, reducing upfront costs and financial risk.
The integration of AI in the energy sector presents a clear pathway to not only overcome existing challenges, but also unlock new opportunities for efficiency, sustainability, and security.
By addressing the issues of data quality, system integration, cybersecurity, regulatory compliance, workforce development, and cost, AI paves the way for a more resilient and flexible energy infrastructure.
As the sector continues to evolve, the role of AI will undoubtedly expand, driving innovation and supporting the global transition to a more sustainable energy future.
AI Trends to Watch in 2024 and Make Your Energy Management Smarter
The energy sector is on the cusp of a transformative era, with AI reshaping its future. The integration of AI in energy management is poised to redefine efficiency, sustainability, and reliability in unprecedented ways.
Looking ahead, several key trends and innovations in AI are expected to drive significant advancements in the energy industry.
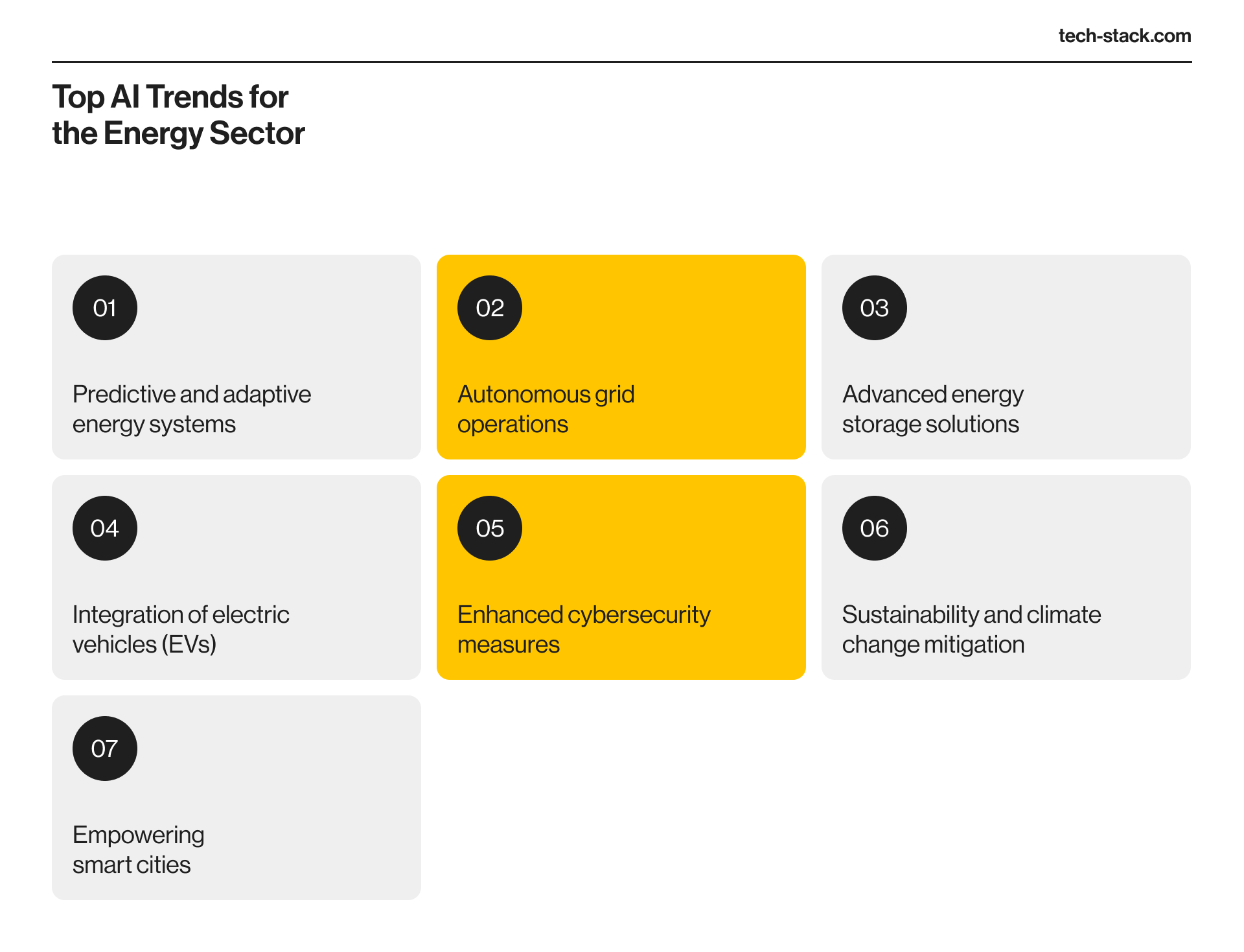
Predictive and adaptive energy systems
AI-powered predictive analytics: The future of AI in energy will see a surge in predictive analytics capabilities, enabling energy systems to forecast demand and supply more accurately. This involves predicting energy consumption patterns and anticipating output from renewable sources like solar and wind, which are influenced by environmental conditions.
Adaptive energy management: With real-time data, AI will facilitate the development of adaptive energy systems that automatically adjust to changing demands and supply conditions. This enhances grid stability and efficiency, reducing waste and optimizing resource allocation.
Autonomous grid operations
Self-healing grids: AI innovations will create autonomous, self-healing energy grids that can detect, diagnose, and repair faults without human intervention. This significantly reduces downtime and improves the reliability of energy distribution.
Decentralized energy resources (DER) management: AI will play a crucial role in managing distributed energy resources, seamlessly integrating them into the grid. This includes optimizing the use of small-scale renewable energy installations and battery storage systems, fostering a more resilient and flexible energy network.
Advanced energy storage solutions
Enhanced battery performance: AI algorithms will optimize charging and discharging cycles for battery storage systems, extending their lifespan and efficiency. Predictive maintenance powered by AI will proactively identify potential issues, reducing the risk of failure and downtime.
Grid-scale energy storage optimization: AI enables more sophisticated management of grid-scale energy storage, balancing supply and demand in real-time and smoothing out the variability of renewable energy sources.
Integration of electric vehicles (EVs)
Smart charging systems: As the adoption of electric vehicles (EVs) accelerates, AI will help manage EV charging demand, prevent grid overload, and optimize charging schedules based on grid capacity and renewable energy availability.
Vehicle-to-Grid (V2G) technologies: AI will facilitate the development of V2G technologies, where electric vehicles can act as mobile energy storage units, supplying energy back to the grid when needed. This bidirectional energy flow enhances grid flexibility and stability.
Enhanced cybersecurity measures
AI-driven security protocols: With the energy sector becoming increasingly digital and interconnected, AI will strengthen cybersecurity measures, using advanced algorithms to detect and neutralize threats in real-time, safeguarding critical infrastructure from cyberattacks.
Sustainability and climate change mitigation
Carbon footprint reduction: AI will optimize energy production, distribution, and consumption patterns to minimize carbon emissions, supporting global efforts to combat climate change.
Renewable energy integration: AI's ability to manage and forecast the output of renewable energy sources will facilitate their integration into the energy mix, accelerating the transition to a sustainable energy future.
Empowering smart cities
Energy-efficient urban planning: AI will play a pivotal role in developing smart cities, optimizing energy usage in buildings and public infrastructure, and enabling efficient urban planning to reduce energy consumption and environmental impact.
The future of AI in the energy sector is marked by innovation and promise. These trends not only highlight AI's potential to revolutionize energy management but also underscore its role in advancing sustainability, efficiency, and resilience.
As AI technologies continue to evolve and mature, their integration into the energy industry will undoubtedly lead to more intelligent, adaptive, and sustainable energy systems, setting the stage for a future where energy is managed in harmony with the needs of both humanity and the planet.
AI Maximizing Energy Efficiency and Sustainability
AI is being increasingly recognized as a game-changer in promoting energy efficiency and sustainability within the energy industry. By utilizing AI, businesses and utilities can optimize energy usage, minimize waste, and facilitate the adoption of more sustainable energy practices. Here's how AI is making a positive impact:
Enhancing energy efficiency
Smart grid optimization: AI algorithms analyze data from smart grids to optimize energy distribution, reducing losses and ensuring energy is delivered where it's needed most efficiently. This leads to a significant reduction in energy wastage and helps utilities manage their resources more effectively.
Demand response management: AI predicts peak energy usage times and implements demand response measures to balance supply and demand. This not only reduces strain on the grid, but also encourages more efficient energy use, preventing overproduction and excessive consumption.
Efficiency in buildings: AI-powered systems in commercial and residential buildings can learn usage patterns and automatically adjust heating, cooling, and lighting for optimal energy use. Such systems can significantly reduce energy consumption and carbon footprints.
Promoting sustainability
Renewable energy integration: AI improves the integration of renewable energy sources into the power grid by forecasting energy production from wind and solar sources. This allows for better planning and reliance on renewable resources, reducing dependency on fossil fuels.
Energy storage optimization: AI enhances the efficiency of energy storage systems, determining the best times to store or release energy based on demand, supply, and energy prices. This optimization supports the use of renewable energy by balancing its intermittent nature.
Resource management: Beyond electricity, AI applications extend to water and waste management, optimizing resource use and reducing environmental impact. For instance, AI can predict water demand and supply, reducing wastage and ensuring more sustainable water management practices.
Smart software for renewable energy
Become eco-friendly, save costs, and reduce your environment impact with custom solutions for renewable energy management. We deliver turnkey products to drive greater sustainability and profitability.
Learn moreAI's contribution to energy efficiency and sustainability is profound and multifaceted. By optimizing energy usage and enhancing the integration of renewable resources, AI puts itself at the forefront of driving the energy industry towards more sustainable and efficient practices.
As AI technology advances, its role in promoting energy efficiency and sustainability is expected to grow, offering promising solutions to global energy and environmental challenges.
Instead of Conclusions
The integration of AI in energy management is a crucial step towards creating more sustainable, efficient, and reliable energy systems worldwide. AI plays a vital role in forecasting, demand response, grid optimization, and managing distributed energy resources. These applications help address the variability of renewable energy sources and ensure a well-balanced energy supply. Innovations such as self-healing grids, advanced energy storage, electric vehicle integration, and enhanced cybersecurity further enhance the transformative potential of AI.
By aligning energy management with environmental sustainability goals and facilitating smart cities, AI contributes to mitigating climate change. AI is critical for advancing the global transition to renewable energy sources. As these technologies continue to evolve, they hold the promise of overcoming challenges and unlocking new opportunities for efficiency and sustainability, making AI-driven solutions indispensable for the future energy landscape.
As a software development company with 10+ years of experience in building custom software products for the energy sector, we know how to power up your energy systems with AI. Contact us to make a leap toward a smarter and greener future.