The Internet of Things (IoT) has drastically revolutionized how companies collect and utilize data, allowing technological advancements to surge with the collection and analysis of data in real-time. There are well over 15 billion connected IoT devices all over the world in 2023, which is projected to double by 2030.
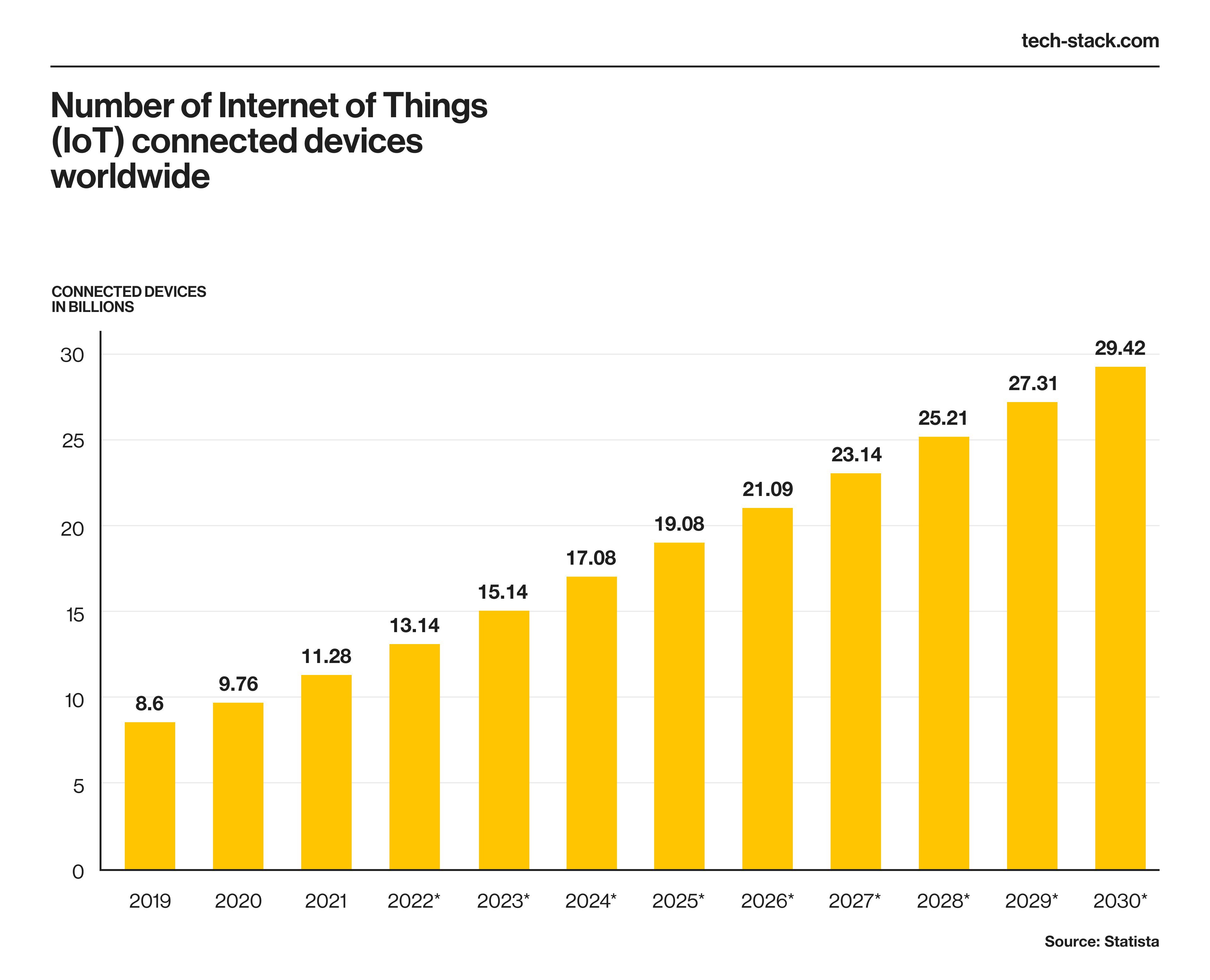
And now, with the trend of digital twins rising within the industry, companies have access to a whole new level of insight into their IoT investments. But what are digital twins? How can they be incorporated into existing IoT networks? What benefits—and challenges—come along with this type of integration?
If these questions have been hovering in your mind lately, then stay tuned, because today we will discuss all of that while also exploring real-life examples of businesses mastering this technology stack. Unleash the power of data insights now!
What Are Digital Twins?
Digital twins are virtual replicas of physical objects that can be used to simulate and analyze their performance in real-world scenarios. They can help manufacturers to understand better how their products work and identify areas for improvement, ultimately leading to more efficient designs and reduced costs.
Did you know that NASA engineers are the OGs of digital twin technology? Back in 1970, they used a twin simulator of the command module and electrical system to save the lives of three astronauts on board Apollo 13. Fast-forward to today, and NASA is still using digital twins, but now they're developing next-gen vehicles and aircraft. This tech has come a long way, and we're here for it!
Digital twins have three main aspects: data acquisition, modeling and application. To do this, they use four powerful technologies: IoT, AI, XR, and Cloud (see picture below). Basically, Digital twins collect real-time data, analyze it with cutting-edge tech, and create digital versions of physical objects. Depending on the application, they apply certain technologies in varying amounts.

When digital twins are integrated with IoT devices, they become even more powerful tools for businesses. IoT sensors can provide real-time data about how a product is working, which can then be fed into the digital twin to create simulations and test out new scenarios. With custom internet of things services, you can test different variables and predict how changes will affect the product's overall performance.
The global digital twin industry is on a skyrocketing path, with a value of $6.5 billion in 2021 and a projected value of $125.7 billion by 2030, according to Allied Market Research. This means it's growing at a rapid pace of 39.48% between 2022 and 2030. Thanks to the rapid growth of IoT and cloud technology, companies are utilizing digital twins to reduce costs and time for product development. Digital twins are a trend that shows no signs of slowing down for the next few years.
Benefits of Integrating Digital Twins with IoT
Integrating digital twins with IoT, or the Internet of Things, can bring about numerous benefits for businesses. One of the most significant advantages is the ability to improve maintenance and repair capabilities. With the real-time data provided by the IoT sensors, businesses can accurately schedule preventive maintenance, monitor equipment performance, and identify potential problems before they occur.
By using digital twins in tandem with IoT sensors, you can also enhance:
- Maintenance and repair capabilities
By combining the power of predictive maintenance with the real-time insights provided by IoT sensors, businesses can optimize maintenance schedules and lower maintenance costs.
By identifying problems early on, businesses can schedule maintenance and repairs during planned downtime, minimizing the impact on production schedules and reducing the cost of repairs. Additionally, digital twins allow for remote monitoring and diagnostics, enabling technicians to identify potential issues and troubleshoot problems from anywhere in the world.
Digital twins with IoT also allow you to track the performance of the assets over time. By collecting data on usage, wear and tear, and other factors, businesses can improve asset management and optimize maintenance schedules. For example, Trenitalia, the main Italian service operator, saved 8-10% on maintenance by adopting IoT and digital twins. This helped not only save money on repairs and maintenance, but also reduce the risk of unexpected downtime, ensuring that lines continue to operate smoothly and efficiently.
- Risk assessment and production time
With digital twins, companies can create a virtual replica of their production process and test it for any potential risks or failures beforehand. This helps engineers identify any problems and come up with mitigation strategies, reducing the risk of costly and time-consuming production errors.
Digital twins are also instrumental in speeding up the development of new products. By simulating the production process, designers can analyze various design options and identify the most efficient and cost-effective ones, saving both time and money.
- Resource allocation and optimization
When combined with IoT sensors that continuously gather data on various aspects of machine performance and production processes, digital twins can provide valuable insights that can help businesses make informed decisions about resource allocation and optimization.
For instance, digital twins can help businesses optimize their production processes by providing insights into factors like material utilization, energy consumption, and machine maintenance. By analyzing real-time data from IoT sensors installed on machines, digital twins can identify inefficiencies and recommend ways to improve equipment utilization, reduce waste, and minimize downtime.
Challenges of Integrating Digital Twins with IoT
Integrating digital twin technology with Internet of Things (IoT) systems can bring about numerous benefits, including real-time monitoring, better predictive analytics, and improved decision-making capabilities. However, there are also challenges that must be addressed in order to integrate these technologies effectively.
- Security and privacy concerns
Digital twins generate large volumes of data, ranging from machine-related information to critical business insights. This information is exceptionally valuable and requires safeguarding against external and internal threats. A cyber-attack on a digital twin could expose trade secrets, intellectual property, and sensitive customer data, endangering a company's reputation and bottom line.
In addition to security concerns, privacy is also a crucial challenge when integrating digital twins with IoT. The data generated by digital twins can contain personal information, such as employee and customer data, which must be protected by privacy laws such as the GDPR and CCPA. Without adequate privacy measures in place, organizations could face significant legal and financial repercussions.
To address these challenges, companies must adopt robust security and privacy policies to protect their digital twins. These policies must encompass not only technical safeguards such as encryption, firewalls, and intrusion detection systems but also human factors such as employee training and awareness programs.
- The need for specialized expertise and training
One of the challenges facing the integration of digital twins with IoT is the need for specialized expertise. The use of digital twins requires knowledge in areas such as software engineering, data science, and automation.
The successful implementation of digital twins requires a team that is well-trained on the technology and its workings. With the right software development partner who is well-trained and has hands-on experience with IoT and digital twins, you can unlock the full potential of digital twin technology to gain valuable insights, optimize operations, and drive innovation.
- Scalability issues
While digital twins have enormous potential for operational efficiency and cost savings, difficulties in composability have slowed their adoption. This challenge only increases when attempting to scale digital twins to thousands or even millions of devices.
The problem lies in the fact that, with so many devices generating and transmitting data, engineers struggle to integrate the various methods through which equipment and sensors collect, process, and format data. Even when this data is integrated, it can be difficult to make sense of, particularly if there are inconsistencies in how it is recorded, stored, and analyzed.
To overcome these challenges, companies must develop common standards and reference frameworks to facilitate simple data interchange. This would make it easier for devices to communicate with one another, regardless of the manufacturer or underlying technology.
Examples of Digital Twins and IoT Integration
Digital twins have been increasingly integrated with Internet of Things (IoT) technology to create smart systems that can monitor and predict performance, optimize processes, and improve efficiency. Here are some notable examples of digital twins and IoT integrations in various industries:

Digital twins and IoT integration are transforming industries by creating smart systems that can monitor and predict performance, optimize processes, and improve efficiency. From manufacturing to healthcare, energy to transportation, the potential use cases for digital twins and IoT integration are seemingly endless. As technology continues to evolve, we can expect to see even more innovative applications of digital twins and IoT.
Techstack Case
As a software services company with 9+ years of experience, we have already built IoT solutions powered by digital twins for agrotech, renewable energy, manufacturing, and e-commerce. Let’s take a closer look at how we harness the power of digital twins for manufacturing:
Data Acquisition: The first step in implementing a digital twin is collecting data from various sources—think sensors, IoT devices, machinery, and relevant data points. This data includes everything from machine performance to quality control parameters.
Data Integration and Modeling: Once the data is acquired, it needs to be integrated and transformed into a digital representation of the physical manufacturing system. This involves creating a virtual model that closely resembles real-world manufacturing processes and assets.
Real-time Monitoring and Control: The digital twin continuously receives real-time data from the manufacturing processes. This data is compared with the digital model to create a virtual representation of the system's current state. Manufacturers can identify potential issues by comparing the real-time data with the digital twin, monitoring performance, and predicting outcomes. Real-time monitoring allows for timely interventions to prevent failures, optimize production, and improve quality.
Performance Analysis and Optimization: The digital twin can analyze historical and real-time data to provide insights into the performance of the manufacturing system. It can identify bottlenecks, inefficiencies, and areas for improvement. It can analyze the data, identify areas for cost reduction, and enhance productivity. The digital twin can simulate "what-if scenarios" to evaluate the impact of changes in the physical system.
Predictive Maintenance: Digital twins enable predictive maintenance by continuously monitoring the condition and performance of machines and equipment. By analyzing real-time data and comparing it with historical patterns, the digital twin can predict potential failures or maintenance needs. This allows manufacturers to schedule maintenance activities proactively, minimizing unplanned downtime and optimizing maintenance costs.
Decision Support: The insights the digital twin provides can support manufacturers’ decision-making processes. By taking a comprehensive view of the system's performance, managers and engineers can make informed decisions to optimize operations, improve product quality, and enhance overall efficiency.
Successful implementation of digital twins necessitates a sturdy infrastructure for acquiring, storing, and processing data. It also calls for the efficient integration of various technologies, including IoT, data analytics, and modeling techniques. Moreover, cross-functional integration among different departments within the manufacturing company is a vital factor that determines the achievement of implementing effective digital twins.
Best Practices for Integrating Digital Twins with IoT
At Techstack, we recommend following these practices for integrating digital twins with IoT to avoid common pitfalls and ensure a successful outcome that delivers tangible results for your business.
1. Define clear business objectives and use cases
The first step in a successful integration is to define clear business objectives and use cases. This will help you identify the key performance indicators (KPIs) that will be used to measure success and ensure that the integration delivers value to your organization.
2. Identify and prioritize data sources
Digital twins rely on data to operate effectively, and integrating IoT data sources can be overwhelming. It is crucial to identify and prioritize data sources based on their relevance to your business objectives.
3. Collaborate with cross-functional teams
Collaboration is vital in any integration project, but it is even more critical when integrating digital twins and IoT. Cross-functional teams should be involved in the decision-making process to ensure that the integration aligns with the needs and goals of each department.
4. Adopt a scalable and flexible platform
Integrating digital twins with IoT involves large volumes of data and requires a platform that is scalable and flexible. We build solutions that can handle the growth of your data and support a variety of data sources and analytics tools.
5. Invest in data security and privacy
IoT devices and digital twins produce sensitive data that must be handled with care. It is essential to have robust data security measures and protocols in place to protect your data and ensure compliance with regulations.
6. Leverage software and hardware tools
Software tools like MATLAB, Simulink, AWS IoT, IBM IoT, and Microsoft Azure IoT Hub support the development and implementation of digital twins. They allow us to create models replicating real-world behavior and integrate sensor data. Hardware tools such as sensors, actuators, gateways, Raspberry Pi’s PCBs, and controllers from leading tech providers like STMicroelectronics, Texas Instruments, and Silicon Labs allow us to collect and transmit data.
These tools optimize asset management, enable efficient operations, and facilitate smarter decision-making through digital twin integration with IoT devices.
Summing Up
In today's increasingly data-driven environment, digital twins provide companies with an almost unparalleled level of insight into their products and processes. With a digital twin, teams can model, simulate, and monitor everything from product design and performance to supply chain operations and customer behavior. By doing so, you can better test, optimize, and improve your business offerings to maximize value across the entire ecosystem.
But, of course, implementing digital twins is not a one-size-fits-all solution. To reap the greatest benefits, you must carefully consider their unique goals and pain points and select a digital twin strategy that aligns with your needs. This requires a deep understanding of the data you’ll need and the specific processes they'll be simulating. Let’s find out how digital twins can power up your business and help you accelerate production time, quality, and cost. Book a free kick-off meeting with our IoT experts!