Despite substantial financial investments in US healthcare in recent years, dissatisfaction looms large.
In a national poll, 60% of surveyed US adults awarded the American healthcare system grade C or below, citing high costs, profit-driven priorities, and insurance inaccessibility as the primary culprits. Behind the scenes, medical workers are also grappling with shortages and burnout in a system in dire need of reform.
Amid such challenges, cloud-based healthcare data analytics could be the ally medical executives need to address soaring costs, professional burnout, operational inefficiencies, and inaccessibility.
This article for decision-makers builds on Techstack’s experience in healthcare solutions and big data development services. Here, our team will explore
- How cloud-based solutions work to transform raw healthcare data into actionable insights
- The four types of data analytics and how they integrate with healthcare
- Existing Big Tech solutions for cloud-based analytics
- The results you can expect from cloud-based data integration, and tips for doing it well
By the end, you’ll have a clear view of how cloud-based healthcare analytics works and what it can offer your organization.
Introduction to Cloud-Based Healthcare Data Analytics
First, let’s be clear: data analysis in healthcare means big data analysis. What’s more, while healthcare data analytics deals with the complexity and diversity common to all big data, it has its own unique challenges due to privacy regulations and the legal and ethical aspects of healthcare data storage and processing.
To begin with, healthcare data falls into two main format categories: structured and unstructured. Taken together, their volume is huge: providers generate a staggering 137 terabytes of data daily, with a 47% increase in healthcare information produced every year.
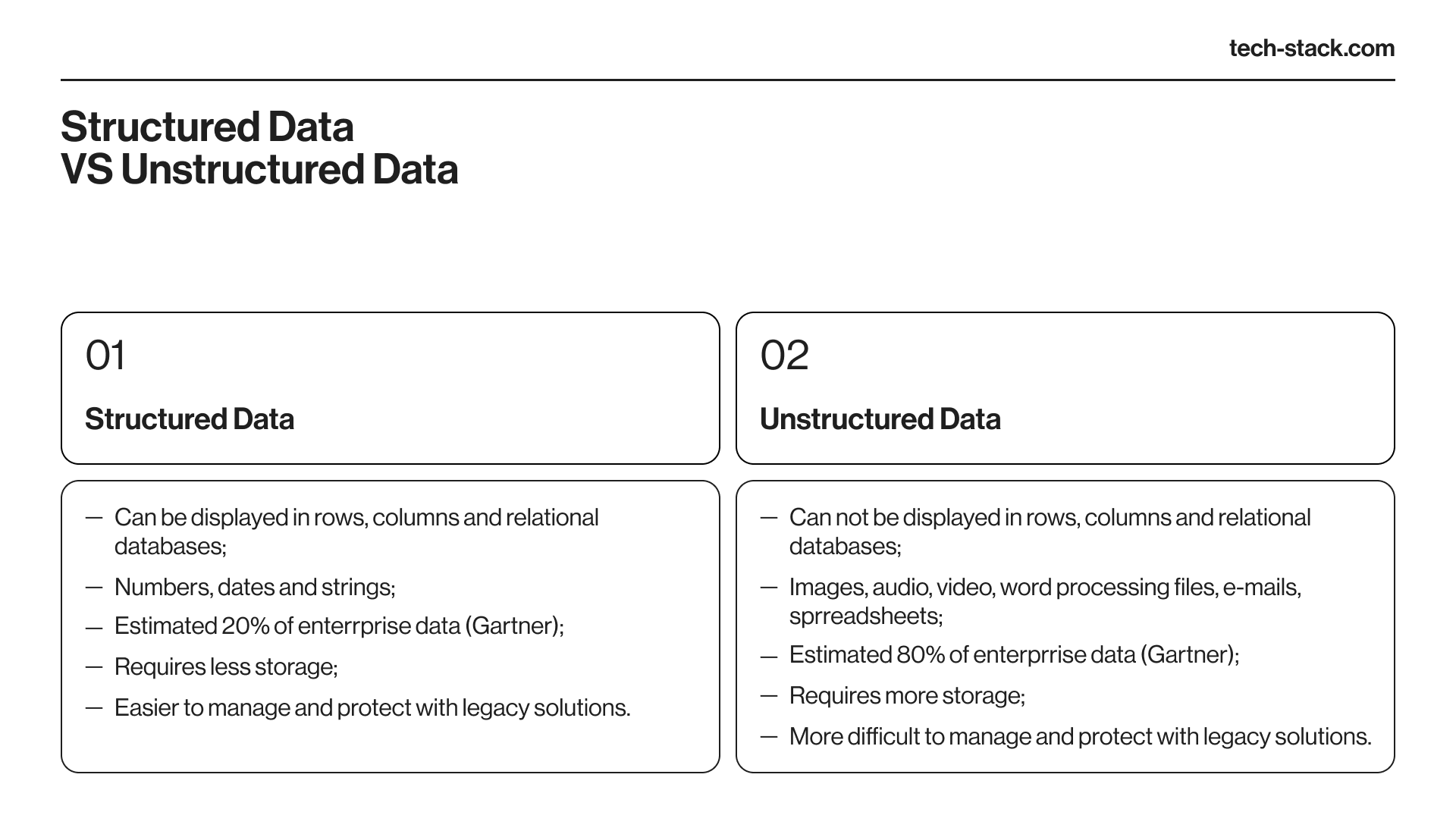
Structured healthcare data is information already stored in a database (or easily transferrable to one) and ready for integration with an analytics tool. Some examples of structured data in electronic health records (EHRs) are
- Patient demographics
- Vital signs
- Lab tests
- Medications
All this data has a fixed structure, meaning it can be easily analyzed with standard statistical or ML-based tools.
Unstructured data, in comparison, is a much tougher processing challenge. Examples of unstructured data in EHRs and other sources are
- Clinical notes
- Surgical records
- Radiology reports
- Pathology reports
- Information from wearables
- Medical images
Unstructured data is diverse, but it’s also huge. Medical imaging alone accounts for around 80% of a healthcare organization’s total clinical content, with file sizes ranging from 15 megabytes for, say, a chest X-ray to 300 megabytes for a 3D mammography file. Digital pathology images—computerized versions of images from microscope slides—occupy around 3 gigabytes.
So, the images are large. And just like clinical notes and discharge summaries (with their typical grammatical errors, ambiguities, and abbreviations), they contain hard-to-extract but critical information. The same goes for data from mobile health apps and medical wearables like heart rate monitors, ECG monitors, and ICDs. These patient-generated insights exist in an EHR in unstructured form only—if they can be transferred there at all. Telehealth data and information from care providers outside the health system are also typically unstructured.
In short, medical data is like pieces of a puzzle that are fractured, uneven, and spread all around the room (or the hospital). Assembling the puzzle (i.e., leveraging your data) means sorting out the pieces, organizing them into groups, making them accessible, establishing a place where the pieces can be safely stored and assembled, and often finding an assistant to help with all these tasks.
And this is what cloud-based data analytics is all about.
The role of technology in health data analytics
Since there are so many pieces of data in the healthcare puzzle, the first challenge is storage, or where to put them all. Modern cloud storage solutions offer the answer: a large, safe, affordable, scalable, and accessible data repository.
Once these data fragments have been gathered in the cloud, the spotlight turns to classification and labeling—a critical step to making data more accessible and usable. Classification involves categorizing data into distinct groups based on predefined criteria such as origin (hospital, lab, research institution, wearable device) and type (treatment plan, patient info, test results). Labeling, in turn, assigns meaningful tags to these data pieces—for example, symptom, diagnosis, or medication.
Storing, classifying, and labeling structured data are relatively easy tasks, but doing the same for messy, unstructured healthcare data takes some serious tech muscle. This is the realm of AI with deep learning, image recognition, and NLP processing, along with complex data formats like ICD-10 and SNOMED.
Once you've cleared these hurdles, you can create data-rich, user-friendly reports that are ready to use in advanced healthcare data analysis and data-driven decision-making.
The Power of Data Analysis in Healthcare
Given the complexity of the healthcare system and its constant striving for cost-efficiency, healthcare data analysis can be a game-changer. When properly implemented, data analysis makes patient care smoother, treatments smarter, and predictions sharper.
Still, not all data analysis is the same. Broadly speaking, there are four types of healthcare data analytics, ranging from descriptive analytics (the easiest and most common) to prescriptive analytics (the most complex). As analytics gets more complex, technology and investment go up. Understanding the differences and overlap between each type is key to judging where your best investment lies.
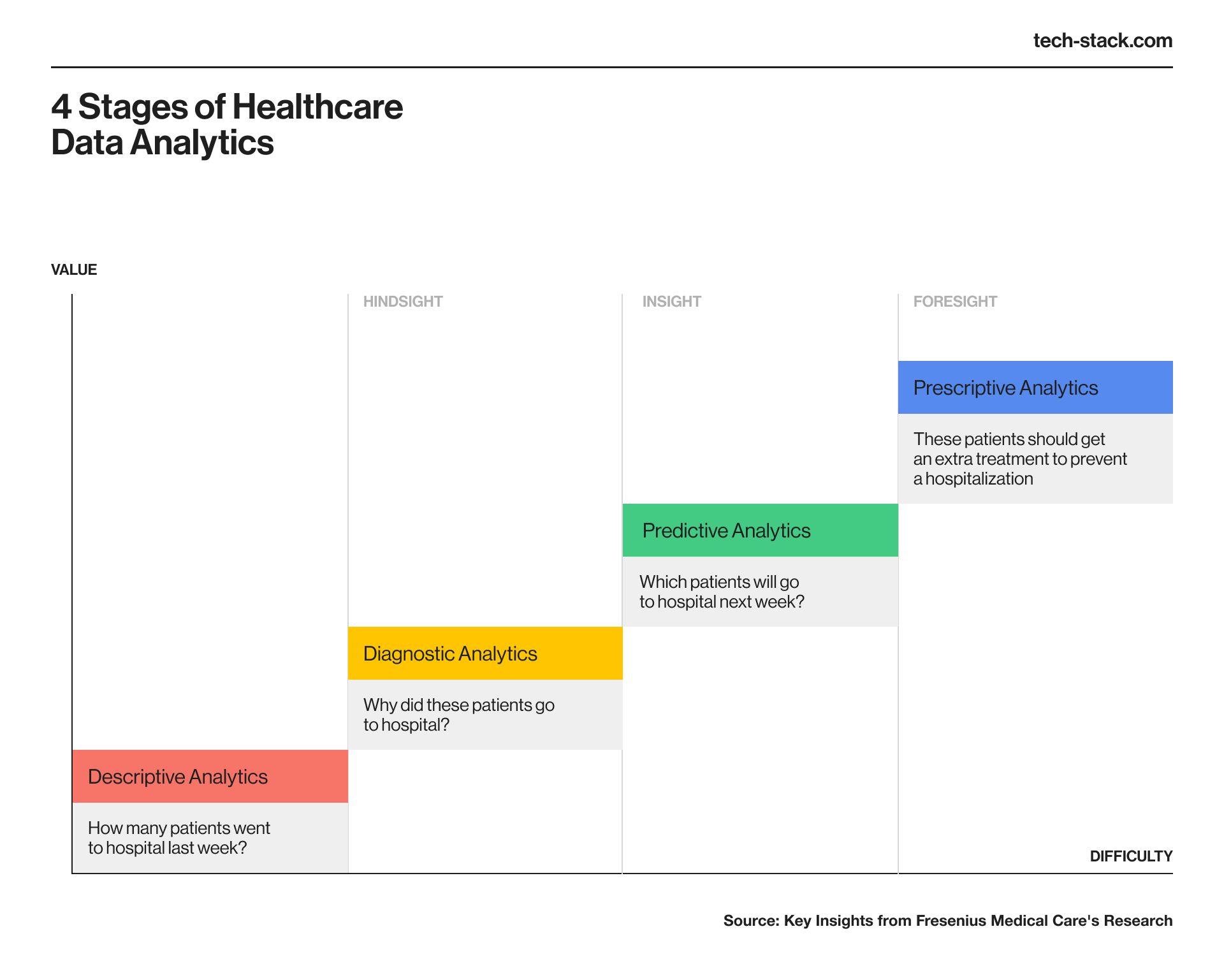
1. Descriptive analytics: What's going on right now?
Descriptive analytics forms the easiest type of data analysis. It examines historical data and provides reports based on this information.
In practice, healthcare organizations can use descriptive analytics to determine, for example, how contagious a virus is by analyzing the number of positive tests during a specific period. Another application is understanding when a hospital is busiest. By analyzing past admission data, clinics can boost staff coverage at peak times and ensure they treat everyone without burning staff out.
2. Diagnostic analytics: Why is it happening?
Diagnostic analytics is essentially detective work. Like descriptive analysis, diagnostic analysis works with past data, but instead of focusing on “what,” it asks “why.” It drills down to what's causing issues, and this insight helps doctors, nurses, and other staff make better decisions.
For example, diagnostic analytics can be used to sort through specific symptoms, find similarities, and diagnose patients faster. For example, suppose a clinic sees many patients returning after being treated. Diagnostic analysis of historical data can highlight the main reason—perhaps new symptoms, incorrect medication prescription, or human error in treatment.
3. Predictive analytics: What will happen next?
Health data analysts use techniques like data mining, statistical modeling, and machine learning to glimpse the future. This work uses clean, sorted, and structured historical data to train models and define patterns and trends. Some possible use cases of predictive analytics are
- Calculating the likelihood of specific complications
- Assigning risk scores for a given condition to particular groups of patients
- Identifying the likelihood of hospitalization
- Predicting the number of patients who will prefer home therapy to a clinical stay
- Forecasting the average revenue per patient visit in the next month or quarter
- Planning the number of additional staff needed for the winter season
Predictive healthcare analytics requires more advanced tools, like the ones leveraging AI.
4. Prescriptive analytics: What’s the best course of action?
While predictive analytics attempts to describe the future, prescriptive analytics tells you the best course of action to take in that scenario. It’s like a personal advisor for making smart choices. By diving into predictive data, scientists determine the factors that can be changed for the better and come up with prescriptions for positive outcomes. This advanced level of analytics allows you to make recommendations on healthcare delivery and process optimization.
In a hospital, prescriptive analytics can suggest the best treatments for patients based on what worked well in the past. Prescriptive analysis can also recommend optimum staffing levels, advise advanced hygiene measures in time for seasonal outbreaks, suggest when home treatment may be better than hospital admission, and much more.
Both predictive and prescriptive analytics unlock the power of your organization’s data, helping you step into a future of precision, efficiency, and patient-centric care. Still, this level of work comes with a heavy price tag. Healthcare data analysts commonly focus on descriptive and diagnostic data analysis, as they don’t require advanced technical tools.
But no matter what level of analysis you’re at, your data has to be properly organized. And that brings us back to the cloud.
Cloud-Based Solutions for Medical Data Analysis
We’ve already seen how cloud storage suits the huge volumes of data generated by healthcare organizations. But there are other reasons that make the cloud ideal for medical data analysis:
- Easier sharing and integration with healthcare analytics tools
- Scalability, which is critical for organizations when it comes to applications like EHRs that grow exponentially
- Compatibility in healthcare contexts like telehealth and remote care
Let’s take a look at how three major cloud providers are ushering in a new era of cloud-based solutions for healthcare. This will help inform your decisions on the most suitable choice.
Microsoft Cloud for Healthcare
Microsoft's cloud-based platform, Azure, stands at the heart of a myriad of revolutionary AI and IoT solutions. Microsoft's offering centers on making the vast trove of healthcare data analytics-ready.
At the heart of this transformation is Microsoft Fabric, an AI-powered platform that integrates seamlessly with Azure. Fabric serves to organize and normalize healthcare data, ensuring it becomes accessible and primed for cloud-based analytics and AI applications.
Another prominent product is the Azure Health Bot. This AI-driven solution—essentially conversational AI for healthcare—enables more personal and efficient patient interaction and triage and generates valuable data for medical data analysts. Learning from interactions, the bot's capabilities reach a deeper understanding of patient preferences and concerns, further enhancing the value of the data gathered.
Microsoft's commitment to healthcare analytics extends to Text Analytics for Health, a prebuilt feature within Azure AI Language. Using machine learning, Text Analytics can extract and label relevant medical information from unstructured texts. This cloud-based service is still under development and can’t be deployed at full scale, but for now, it allows extracting Social Determinants of Health (SDOH) and ethnicity mentions.
The industry has been quick to implement this and other Microsoft technology, leading to groundbreaking solutions. Microsoft’s HoloLens 2 has found valuable applications in medical training and acute and critical patient care. Connecting Azure with the Medical Open Network for Artificial Intelligence (MONAI) has also had excellent results for 3D brain tumor segmentation.
Amazon Web Services
Amazon SageMaker, a robust platform tailored for healthcare and the life sciences, is at the forefront of AWS's healthcare revolution. This service empowers organizations to build, train, and deploy ML models at scale, resulting in deeper and more accurate healthcare analytics. The platform has a wide range of applications, including extracting and analyzing data from documents, spotting anomalies in medical images, detecting suspicious claims and transactions, and labeling training data for different use cases.
Another AWS offering is Amazon HealthLake, a HIPAA-eligible service designed to aggregate, organize, and structure health data. It provides Fast Healthcare Interoperability Resources (FHIR) APIs and addresses the challenge of disparate and unstructured healthcare information, providing a foundation for advanced analytics and machine learning applications.
AWS's impact in healthcare extends beyond its platform through strategic collaborations that accelerate drug development, clinical research, and diagnostic capabilities.
- AWS’s partnership with Pfizer showcases how the platform’s advanced cloud-based solutions can help at different stages of drug development and clinical trials.
- Cooperation with Philips highlights how AWS's cloud services can help deliver innovative healthcare solutions through the Philips HealthSuite Platform, which gathers and processes information from various sources like EHRs, wearables, insurance companies, and healthcare organizations.
- AWS's partnership with a Munich-based leukemia lab shows the potential of cloud-based machine learning. The lab uses Amazon SageMaker to achieve breakthroughs in leukemia diagnosis, demonstrating the practical impact of AWS's advanced analytics capabilities in the medical field.
The Techstack team also opted for AWS when building a heartbeat screening module for a US-based healthcare partner. A serverless microservice architecture on AWS gave us a scalable and reliable way to digitize, store, analyze, and transmit heartbeat data. As a result, data analytics for our healthcare partner became straightforward, efficient, and streamlined.
Techstack Case
The Techstack team also opted for AWS when building a heartbeat screening module for a US-based healthcare partner. A serverless microservice architecture on AWS gave us a scalable and reliable way to digitize, store, analyze, and transmit heartbeat data. As a result, data analytics for our healthcare partner became straightforward, efficient, and streamlined.
Read full caseIBM
IBM's healthcare initiatives are built around the IBM Cloud Pak for Integration, which includes IBM App Connect, a powerful tool that facilitates the implementation of FHIR and HL7 standards. IBM Cloud Pak empowers healthcare providers to connect disparate systems, streamline workflows, and improve operational efficiency.
Another notable IBM product for healthcare is the Knowledge Accelerator for Advanced Analytics Services (KAaaS). This industry-specific vocabulary for healthcare providers serves as a central knowledge base for different departments and business operations. It also includes healthcare regulations, standards, and customizable reference data.
IBM's offering in healthcare analytics hasn't been without setbacks. Its health-focused conversational AI technology, Watson Health, was designed to revolutionize healthcare, helping with sorting, categorizing, and finding patterns in vast unstructured oncology data, facilitating clinical research, and analyzing medical images. But in 2022, Watson Health was sold for parts: a private equity firm bought part of Watson with all the data and analytics tools for healthcare data.
So, while other tech giants are increasing their share in advanced healthcare solutions, IBM gave up on its initiative because of serious strategic mistakes and financial loss. Nevertheless, Watson’s healthcare data still has significant value (over $1 billion, to be precise), and medical companies can still use the capabilities of IBM Cloud to develop healthcare solutions.
To sum up, medical data analysts use cloud-based solutions in areas such as
- Data warehousing
- Big data analytics
- Predictive analytics
- AI-based medical imaging analysis
- Business intelligence
- Data visualization
Employee training plays an important role in getting your organization up to speed. As AI- and cloud-based solutions become more prevalent, medical professionals need to upgrade their technology skills to ensure everyone gets the most out of their investment.
Key Benefits of Using Data Analysis Tools in Healthcare
Up to now, we’ve mentioned some general applications of cloud-based data analysis in healthcare, but what’s really in it for you? Through nine years of software development for the healthcare industry, we’ve seen the benefits of cloud-based analytics firsthand. Here’s what you can expect.
Data-driven insights at scale
One of the primary benefits of data analytics for healthcare is more and better data-driven decisions. By leveraging insights from advanced analytics tools in the cloud, decision-makers can make better strategic choices, optimize resource allocation, and improve efficiency. The inherent scalability of cloud storage and tools means you can store all your data in one place and adjust your analytics capabilities based on demand. The cloud also frees up your on-site storage for your most recent data.
Improved patient care through personalization
Healthcare analytics helps identify patterns and trends in patient data that lead to better patient interactions and outcomes. Through predictive and prescriptive analytics on the cloud, healthcare analysts can anticipate potential health issues, personalize treatment plans, and intervene proactively. This also contributes to preventive and personalized medicine.
Flexible operations and cost-efficiency
IT consulting company Veritis suggests that 83% of healthcare providers have already turned to the cloud for their operations, as it offers the cost-efficiency they seek. With applications ranging from supply chain management to resource allocation, healthcare analytics tools in the cloud can identify bottlenecks, streamline workflows, and highlight unnecessary costs. The flexibility of cloud platforms also ensures that healthcare organizations can adapt their infrastructure based on evolving operational needs and pay for only what they use.
Enhanced health management through data integration
Healthcare analytics facilitates comprehensive health management by analyzing high quantities of data from different institutions (clinics, labs, insurance companies) and sources (EHRs, records, wearables, telehealth solutions). Once datasets have been integrated, cleaned, and structured to be AI-ready, healthcare organizations can identify at-risk citizens, design targeted interventions, and allocate resources where they are most needed.
A secure environment for healthcare data
Cloud providers invest heavily in security, advanced data protection, and compliance with regulations such as HIPAA, giving you a reliable environment for sensitive patient data. Access controls and advanced authentication can also be easily customized in the cloud. Finally, the cloud ensures data backup and disaster recovery—tasks that are much more complex on-premises. In summary, the benefits of migrating your healthcare analytics to the cloud are huge. But so are the challenges. Working with a competent software development partner with expertise in the healthcare domain is the best way to overcome them, but if you choose to go it alone or want to prepare better, here are Techstack’s tips on leveraging cloud-based solutions.
Tips on Efficient Use of Cloud for Data Analytics in Healthcare
The first step to successfully implementing cloud-based analytics is establishing a robust data framework. This task involves defining data ownership, access controls, and data lifecycle management protocols. Here are our tips for getting it right:
- Leverage your cloud provider’s identity and access management (IAM) tools to assign specific permissions to individuals or roles within your healthcare organization.
- Define protocols for data creation, storage, and deletion to ensure regulation compliance. Cloud providers typically offer tools for data lifecycle management that help automate data retention and archival processes.
- Use tools for anonymizing and de-identifying healthcare data, such as FHIR Tools on Azure or Apexon’s solution on AWS. With these, healthcare data analysts can remove personally identifiable information (PII) from datasets without impacting their analytical value.
- When utilizing cloud-based analytics, ensure patients are informed about how their data will be used and seek explicit consent. Cloud platforms often provide mechanisms for tracking and auditing data access, enhancing transparency and accountability in analytics.
The next challenge to address is cloud-based data recovery. The main goal is to ensure that your workload can be brought back online or continue functioning with minimal downtime in the event of a disaster. Cloud providers offer different disaster recovery strategies, ensuring critical healthcare data can be swiftly recovered when required.
Last but not least, the ultimate goal of data analytics is to receive data-driven insights in healthcare and put them into action. The cloud is a perfect place to access and collaborate on data, but you’ll also need advanced visualization and reporting tools to communicate analytical findings. While getting the results of data analysis to decision-makers is the last step, it’s also critical: only with clear and user-friendly visualization will healthcare data analytics serve its goal.
Conclusion
In a healthcare sector plagued by challenges like rising costs and employee burnout, the need for transformative solutions has never been greater. Analytics unlocks the power of healthcare data and paves the way to more efficient resource allocation, optimized workflows, and personalized patient care.
Cloud platforms are a logical choice for leveraging both structured and unstructured healthcare data. They offer scalability, accessibility, and cost efficiency, and include a wealth of tools for data storage, processing, and analysis.
Yet storage and processing power are just two pieces of the puzzle. Medical professionals, too, need the technical skills to efficiently utilize the intricacies of advanced analytics, and organizations need reliable technical partners who can help implement change at scale.
Whether you’re planning your cloud-based healthcare solution, moving your operations to the cloud, or building your analytics capability, Techstack has the expertise to help. Get started on your journey by learning more about our experience in healthcare or contacting the Techstack team for a free exploratory conversation.