Over the past decade, advancements in sensor technologies, smart meters, satellite imaging, and other monitoring systems have led to an explosion of data in the energy industry. From real-time transmission line sensor data to historical weather pattern information, energy companies now have huge datasets at their fingertips. At the same time, innovations in data processing, machine learning, and artificial intelligence are making it possible to gain meaningful insights from all of this data.
As a result, data-driven decision-making is becoming integral to success in the energy sector. By harnessing big data analytics, energy companies can optimize asset performance, forecast electricity demand, integrate renewable energy sources, and make operations safer and more efficient.
McKinsey estimates that big data analytics could reduce costs in the electricity value chain by as much as 25%.
Big data technology refers to advanced analytics techniques and tools that can process high volumes of diverse energy data to uncover patterns, insights, and opportunities.
Some key capabilities of big data in energy include:
- Handling large, complex energy datasets
- High-speed data processing and analysis
- Data mining and pattern recognition
- Statistical modeling and machine learning
- Visualization of data and results
Utility providers, energy companies, and other stakeholders can apply energy big data solutions to extract value from energy data. This allows them to optimize operations, forecast demand, prevent equipment failures, integrate renewable energy, and ultimately provide affordable, reliable, and sustainable energy.
This blog post will explore how big data is transforming various aspects of the energy industry. We will look at key technologies enabling better data collection and analysis, innovative data management solutions, and real-world case studies of data-driven innovation in the energy sector.
The potential of advanced analytics for energy optimization, smart grid data applications, data visualization, and more will be discussed. Our goal is to showcase the immense opportunities for big data to drive the future of energy.
Big Data Technology in the Energy Industry
Big data technology is rapidly transforming the energy industry by enabling companies to collect, store, and analyze vast amounts of data. This data deluge is providing new insights that can optimize energy operations, improve efficiency, and develop innovative products and services.
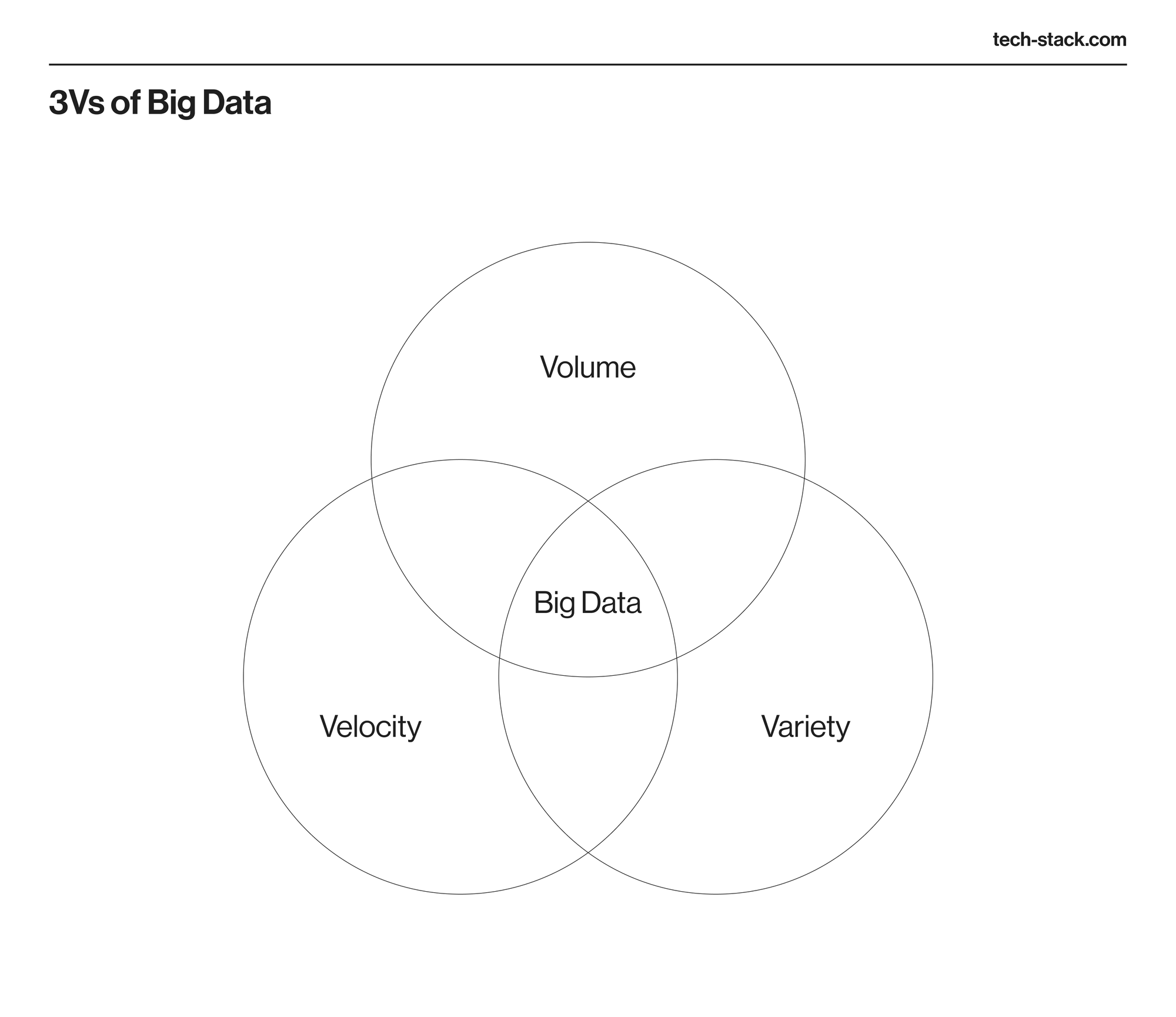
The energy sector generates massive amounts of data from a variety of sources. Smart meters installed in homes and businesses record granular electricity usage data. Sensors embedded in transmission lines, pipelines, wind turbines, and other assets monitor performance and detect issues. Energy trading platforms contain enormous volumes of pricing and transactional data. Weather satellites and sensors collect climate data relevant to energy production and demand.
The volume of big data in the energy industry is staggering. Terabytes of data are generated each second. The velocity at which this data is produced is also immense, with real-time streams from sensors across energy infrastructure. Finally, the variety of structured and unstructured data from disparate sources provides challenges and opportunities for big data energy analytics solutions.
Here are some examples of big data sources and types in the energy sector:
- Smart Meters:
- Advanced metering infrastructure (AMI) data
- Real-time electricity consumption data
- Voltage and power quality data
2. Sensor Networks:
- Industrial Internet of Things (IIoT) sensors in power plants
- Weather sensors for renewable energy generation (e.g., wind and solar)
- Grid sensors for monitoring grid health and stability
3. Energy Generation Data:
- Data from power plants, including fossil fuels, nuclear, and renewable sources
- Solar irradiance and wind speed data
- Data from hydroelectric, geothermal, and biomass facilities
4. Grid Operations Data:
- Grid frequency and voltage data
- Substation and transformer monitoring data
- Power flow and line congestion data
5. Energy Consumption Data:
- Data from residential, commercial, and industrial consumers
- Historical consumption patterns
- Peak load and demand response data
6. Weather and Environmental Data:
- Weather forecasts and historical weather data
- Environmental impact data (e.g., carbon emissions)
- Climate data for long-term energy planning
7. Energy Market Data:
- Market prices for electricity, natural gas, and other energy commodities
- Trading and transaction data from energy exchanges
- Supply and demand data for energy markets
8. Maintenance and Asset Management Data:
- Equipment condition and health monitoring data
- Predictive maintenance data to minimize downtime
- Asset performance data for optimizing resource allocation
9. Geographic Information System (GIS) Data:
- Geospatial data for energy infrastructure mapping
- Location-based data for siting new energy projects
- Land use and environmental data for regulatory compliance
10. Energy Efficiency Data:
- Energy audits and efficiency improvement data
- Building energy management system (BEMS) data
- Energy conservation program data
11. Customer Data:
- Customer demographics and behavior data
- Customer feedback and preferences
- Billing and payment data
12. Cybersecurity Data:
- Data related to cybersecurity threats and incidents
- Network and system security logs
- Anomaly detection data for early threat identification
Success Stories of Data-Driven Energy Providers
Leading energy companies are already seeing the benefits of big data transformation. For example, P&G leveraged sensor data analytics to predict asset failures before they occurred, resulting in massive savings annually.
Such success stories demonstrate the immense potential of data-driven innovation in the energy domain. As more companies embrace data, they will unlock significant value for their businesses while enabling a more sustainable energy future. Here’s one more real-world example of how our software development company helped a solar energy company get the best out of big data.
Techstack Case
In the ever-evolving landscape of renewable energy, our team successfully developed a robust solar energy production platform. The platform was designed with the key objective of collecting and storing solar production energy data from various vendors. Our solution was engineered to be scalable, capable of accumulating data on an hourly basis as well as historical data. We also created a fast and sturdy data storage system that facilitated data aggregation on a weekly, monthly, and yearly basis. Furthermore, the platform was equipped with features for presenting data in diverse ways, providing comprehensive monitoring and analytics based on energy production data by region and inverter statuses.
However, the development process was not without its challenges. One of the primary hurdles was dealing with a vast number of IoT sensors and devices that required APIs. Given the extensive scale of data collection involved, it was imperative to develop an efficient mechanism to manage these APIs. Additionally, the issue of data coming in different formats posed another challenge. The varying data formats necessitated a system that could seamlessly integrate this diverse data into one cohesive platform.
The team addressed these challenges head-on with innovative solutions. To manage the plethora of IoT sensors and devices, we implemented a system of APIs that could efficiently handle the volume and variety of data. To tackle the issue of varying data formats, we developed a process for data normalization. This process ensured that all incoming data, regardless of its original format, was converted into a standard format suitable for our platform. This not only streamlined the data integration process but also enhanced the platform's overall functionality and efficiency.
Advantages of Big Data in the Energy Sector
The energy sector is ripe for transformation through the power of big data analytics. As this industry continues to generate massive amounts of data, organizations that effectively collect, process, and analyze this information will be poised to unlock game-changing insights and innovations. In this section, we'll explore some of the key advantages big data offers across the energy sector.
Optimized asset management
One of the most notable advantages of big data technology is its ability to optimize asset management. Through the analysis of sensor data from equipment such as turbines and transformers, predictive maintenance can be carried out, thereby reducing downtime and costs. This proactive approach enables energy companies to identify potential issues before they result in equipment failure, ensuring uninterrupted operation and greater overall efficiency.
Improved demand forecasting
Big data also plays a crucial role in improving demand forecasting. Advanced forecasting models that leverage weather, pricing, and usage data enable better planning and more accurate prediction of energy needs. This level of precision helps to minimize waste and to ensure that resources are utilized optimally, further contributing to energy efficiency.
Personalized energy management
Personalized energy management is another area where big data technology shines. By analyzing smart meter data, companies can gain insights into customer usage patterns. These insights can then be used to design tailored efficiency programs that help customers manage their energy consumption more effectively. Such personalized interventions not only benefit individual customers, but also contribute to broader energy conservation efforts.
Grid optimization
Grid optimization is a further benefit provided by big data technology for renewable energy software development. By identifying high-load areas and peak demand times, targeted infrastructure improvements can be made. This strategic approach to infrastructure development ensures that the energy grid is equipped to handle demand efficiently, reducing the risk of blackouts and other disruptions.
Integration of renewable energy
The integration of renewable energy sources into the energy mix is another critical area where big data can have a significant impact. Analytics can help predict renewable generation, allowing for the calibration of the grid to accommodate these sources. This capability is particularly important as the world seeks to transition to cleaner, more sustainable energy sources.
Reduced energy consumption
Finally, big data technology can facilitate reduced energy consumption. By providing data-driven insights into usage patterns, it enables the development and implementation of energy conservation initiatives. These initiatives can range from encouraging changes in individual behavior to implementing large-scale efficiency measures in industrial settings.
Energy efficiency recommendations for your customers
Utilize big data to offer customers personalized recommendations on how to increase their energy efficiency and reduce costs. This can include suggestions for optimizing energy consumption and implementing energy-saving technologies. Positioning your renewable energy solutions as a way to achieve cost savings can be a compelling marketing strategy.
Overall, big data technology allows various energy sector stakeholders to make smarter decisions and coordinate their actions, driving systemic efficiency gain
Key Challenges With Energy Data Management Solutions
With the massive amount of data generated in the energy sector, effective data management is crucial and the most challenging aspect of big data development services. An energy data management solution provides capabilities to collect, store, process, analyze, and visualize energy data from disparate sources in a meaningful way. Proper data management in energy delivers several key benefits:
- Data quality and reliability: Removing duplicates, detecting errors, and reconciling data from multiple sources.
- Improved data accessibility: Storing data in compatible formats and making it available to different applications and users.
- Enhanced data security: Managing access permissions and implementing cybersecurity measures.
- Compliance and auditing: Adhering to regulations regarding data governance and retention policies.
- Operational efficiency: Eliminating redundant data collection and enabling data-driven decision-making.
With robust energy data management, utility providers and energy companies can turn raw data into meaningful insights that drive sustainability, cost savings, and innovation.
Collecting and storing energy data
A key aspect of energy data management is collecting and storing relevant data from myriad sources across the energy value chain. This includes:
- Smart meter data on customer electricity usage
- Sensor data from transmission lines, pipelines, and other assets
- Supervisory control and data acquisition (SCADA) systems data
- Weather data from meteorological agencies
- Pricing data from energy markets
- Billing and customer account data
- Renewable energy generation data
This time-series data is gathered in intervals ranging from milliseconds to months. The data management system stores this in databases optimized for time-series data, ensuring efficient writes and reads.
Ensuring data quality and security
With data coming in from multiple sources such as smart meters, sensors, SCADA systems, meteorological agencies, energy markets, and customer accounts, there's a high chance of duplicates, errors, and inconsistencies. These issues can severely affect the reliability of the data and, consequently, the insights derived from it.
The steps involved include:
- Identifying missing, duplicate, or erroneous data
- Flagging, investigating, and fixing bad data
- Validating data relationships and correlations
- Establishing master data management protocols
- Applying security controls and access restrictions
- Encrypting data transmission and storage
- Creating contingency plans for data recovery
High-quality, secured data is essential for reliable analytics and decision-making in the energy sector.
Integrating data from various sources
Data integration is a critical task in data management that involves combining data from various disparate sources into a coherent and unified view. This process is particularly important in industries such as the energy sector, where it can provide valuable insights to optimize the energy value chain.
A key data management task is integrating diverse datasets coming from different devices and sensors into a unified platform. This involves:
- Ingesting structured, semi-structured, and unstructured data
The first step in data integration is the ingestion of structured, semi-structured, and unstructured data. Structured data refers to information with a high degree of organization, like databases or spreadsheets. Semi-structured data, on the other hand, does not conform to the formal structure of data models but contains tags or other markers to separate semantic elements and enforce hierarchies of records and fields within the data. Examples include XML and JSON files.
Unstructured data includes text files, images, audio and video files, emails, social media posts, and more. These diverse data types need to be ingested into the data integration platform using various tools and techniques.
- Resolving semantic differences in data from various sources
Resolving semantic differences in data from various sources is another key aspect of data integration. Different systems may use different terms or definitions for the same concept. For instance, one system might label a field as "customer ID," while another system calls it "client identifier." Resolving these differences requires mapping these terms to a common understanding or ontology to ensure consistency across all datasets.
- Normalizing data units and formats
Data normalization is also crucial. It involves standardizing data units and formats to ensure they are consistent and comparable. For example, dates might be represented differently in different datasets (DD/MM/YYYY vs. MM-DD-YYYY), and these would need to be normalized to a single format.
- Defining common keys to link datasets
Defining common keys to link datasets is another important step. These keys, often called primary keys in database parlance, uniquely identify each record in a dataset. When integrating multiple datasets, it's necessary to identify common keys that can be used to join the datasets together.
- Applying ETL processes
The ETL (extract, transform, load) process is central to data integration. Extraction involves pulling data from various sources. Transformation refers to the process of cleaning, validating, and modifying data to fit into a desired format. Loading is the final step, where the transformed data is loaded into a target database or data warehouse.
- Establishing data governance standards
Lastly, establishing data governance standards is crucial to ensure that the data is accurate, consistent, and usable. Data governance involves setting up rules, policies, and procedures for data management, including access control, data quality checks, and audit trails.
By effectively integrating diverse datasets, organizations can achieve a harmonized view of their data. This unified platform allows for advanced analytics that can uncover deeper insights, leading to more informed decision-making and optimization of processes like the energy value chain. For example, integrated data could reveal patterns in energy consumption, potential areas for efficiency improvements, or opportunities for renewable energy integration.
Data-Driven Transformation in Energy
The energy industry is undergoing a profound transformation driven by the power of data. As energy companies collect and analyze vast amounts of information from smart meters, sensors, and other sources, they are gaining valuable insights to optimize operations, reduce costs, and boost sustainability.
Revolutionizing energy with data-driven approaches
Traditionally, energy companies relied on industry knowledge and intuition to make decisions. But with the advent of big data, they can now take a data-driven approach to glean actionable intelligence. By leveraging analytics and machine learning algorithms, energy firms can uncover patterns, predict usage trends, prevent equipment failures, and customize offerings for consumers.
For instance, utility companies analyze smart meter data to understand energy consumption behaviors. They can then develop targeted energy efficiency programs to help consumers reduce waste. Data is also enabling predictive maintenance of energy assets to minimize downtime.
Improving efficiency and sustainability
Data-driven energy is leading to enhanced efficiency, lower costs, and increased sustainability across the industry. According to Capgemini Consulting, data analytics could help the utility sector save $57 billion per year globally.
By optimizing energy usage based on data insights, firms can reduce overhead costs. Data also unlocks new revenue opportunities, like providing value-added services to consumers. Ultimately, the smarter use of energy enabled by data helps curb emissions and environmental impact.
Final Thoughts
The energy sector stands poised on the brink of a data-driven revolution. As we have seen, big data offers tremendous advantages across the industry, from fossil fuels to renewables. By harnessing the power of data, energy companies can boost efficiency, reduce costs, optimize operations, and make more informed decisions.
The insights unlocked by big data analytics enable everything from predictive maintenance of assets to forecasting renewable energy production. Although data privacy and skill gaps remain challenges, the potential benefits make this a technological wave worth catching.
The message is clear - big data is here to stay and will only grow in importance. The energy sector must be proactive in adopting big data solutions. Companies that embrace data-driven decision-making will gain a competitive edge. Leaders must foster a data-driven culture and invest in analytics capabilities.
For individuals, the path forward involves developing data science skills and keeping up with the latest developments. Conferences, courses, and publications offer opportunities to stay updated on big data in energy. This is an exciting time, as data empowers the industry to work smarter, faster, and more efficiently.The future looks bright, as big data propels the energy sector into a new era of innovation and productivity. Harnessing the power of data is key to overcoming challenges and unlocking the full potential of the industry, and for that, we've got you covered! Just contact us to discuss all the details.