With big data getting bigger annually, many companies are seeking solutions to process and use it for further business analytics. Even though the amount of data is increasing, the latest research suggests that 42% of technologists struggle with sourcing quality data. That’s no wonder, considering the number of channels it comes from. The question is, how can a business prepare for more zettabytes to come?
Understanding the future of big data is essential to plan strategic tech adoption. And that’s what we’re going to explore in this article. First, let’s review the connection between big data and other innovative technologies.
How Big Data is Related to Other Technologies
The use of big data has plenty of benefits for businesses. It helps streamline the decision-making process, provides valuable user behavior insights, improves cybersecurity, and so on. Moreover, it’s a critical part of BI and analytics software that helps businesses measure and manage performance and integrations.
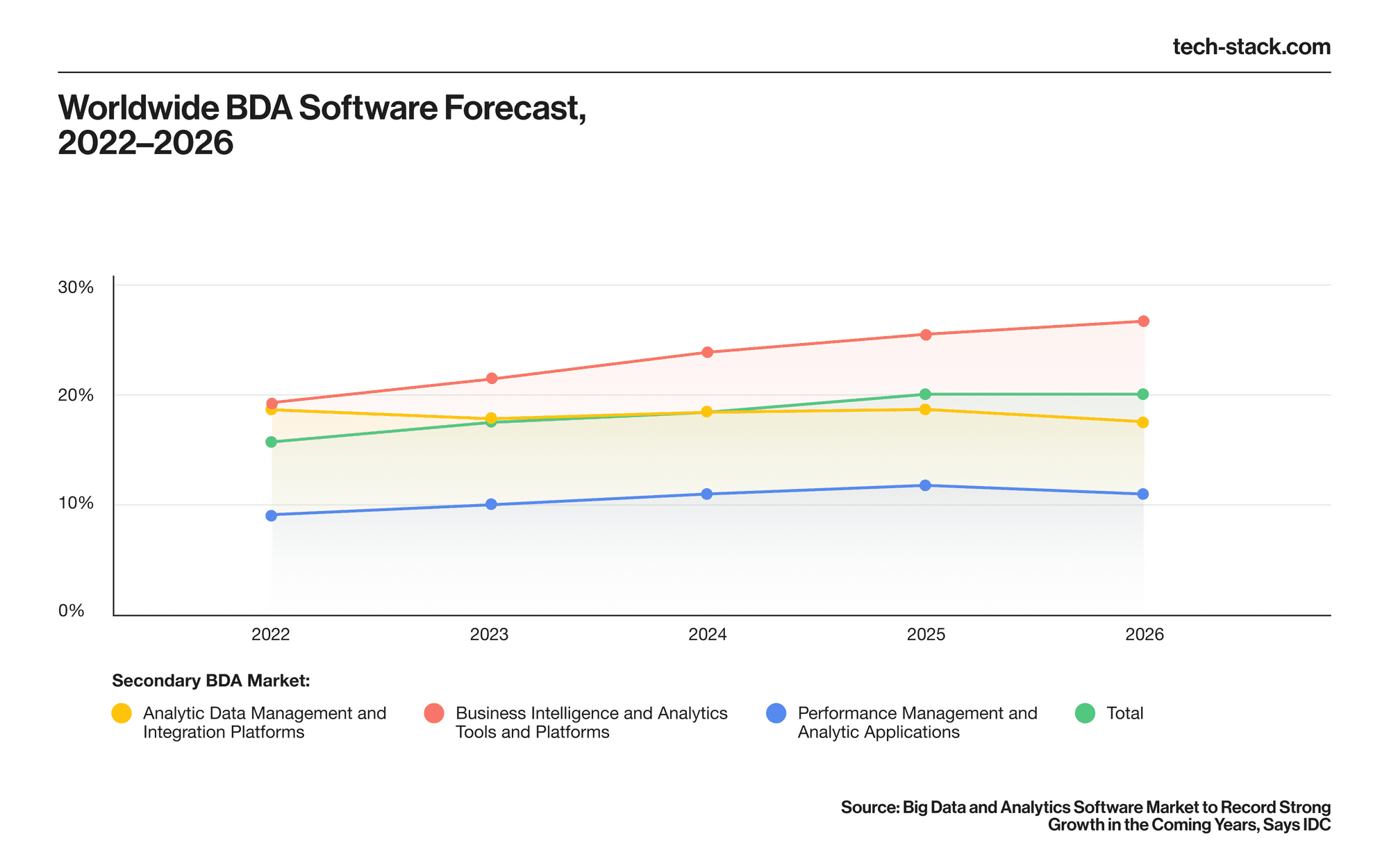
However, the success of data management depends not only on the quality of big data — other technologies must be part of the mix. Here are a few innovative technologies that are connected to big data.
Machine learning and artificial intelligence in big data
Many companies use artificial intelligence and machine learning in various business processes. According to the 2021 McKinsey report, service-operations optimization, product enhancement, and contact center automation are top use cases. The investment is well-justified—73% of businesses consider AI critical to their success.
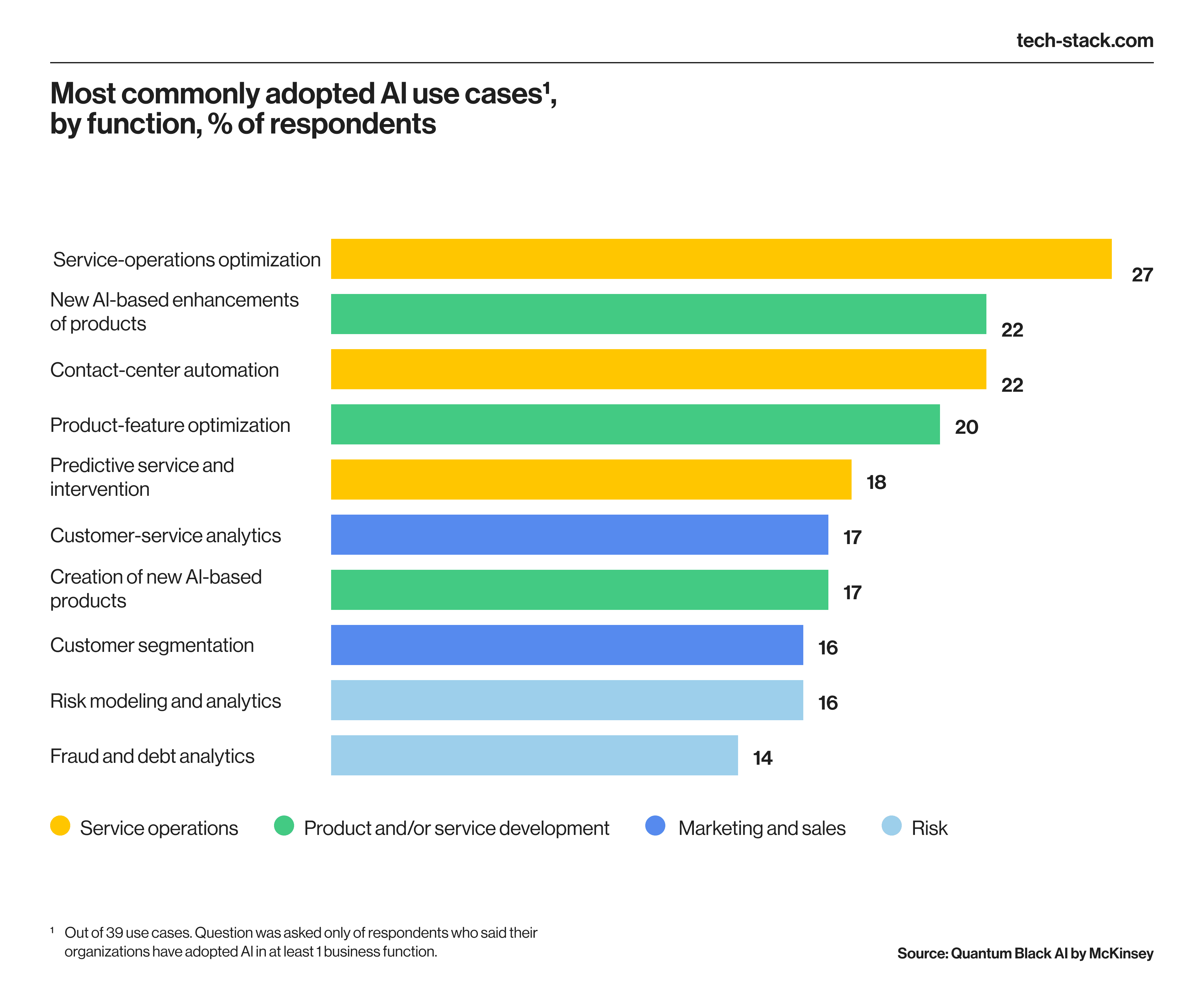
Artificial intelligence, machine learning, and big data are incredibly connected, and these relationships go both ways.
ML automatically processes large volumes of data, which helps scientists extract and structure quality data for further use. Meanwhile, AI solutions need data to create algorithms and build statistical models to analyze data sets. In each case, big data is used to train the model or create new models.
One example of how a company can use big data and machine learning is the case of Amazon Personalize (AP). This customizable API solution helps businesses improve and personalize customer communication through various channels. The company using AP only needs to add its unique data (user-related events and interactions) and customize the model based on this data.
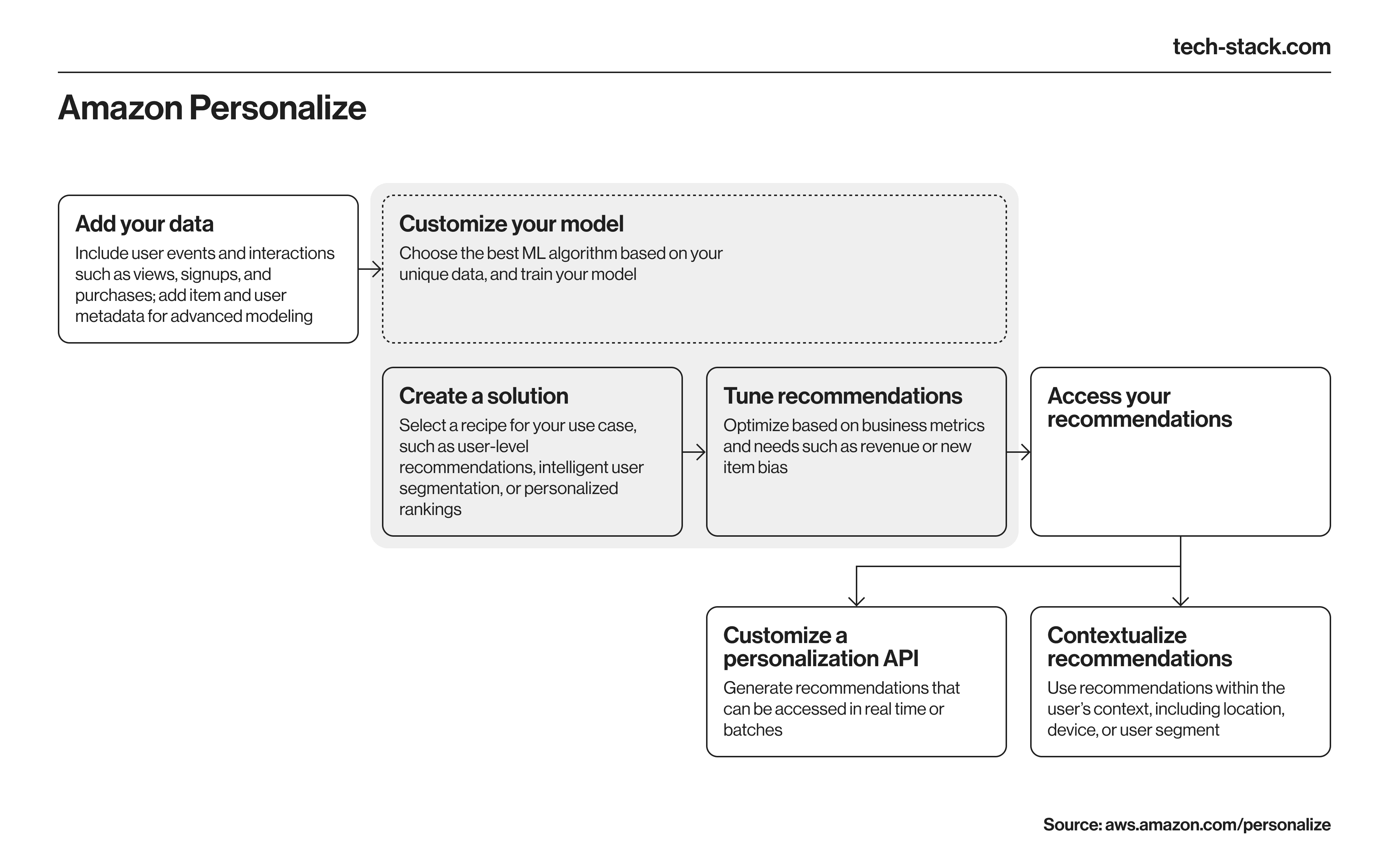
However, engineers must ensure data quality for AI solutions to work. Quality data is necessary for any AI and ML-based product to perform flawlessly and prevent biased results. According to Appen’s report, 51% of the projects owe their success to the accuracy of data sets. And with the current multichannel data scape, data quality is more difficult to obtain.
The Internet of Things and big data
IoT technology is widely used in various industries, such as healthcare and commerce, and this trend is here to stay. The IoT solutions connect the hardware to the internet, enabling the IoT device to collect and transmit data.
In the case of IoT, big data provides platforms and techniques for data analysis, pattern recognition, algorithm creation, and decision-making. Techstack has a case in point that shows their relationships.
Techstack case
Our partners commissioned an agro-tech tracking and aggregation solution for harvesting beans. Its objective was to improve transparency during harvesting, transportation, quality control, and data management and reduce manual work in the field. One of the system components was a mobile app to collect, synchronize, and process data. For the solution to be successful, we created a proper data analysis framework and platform to manage and analyze the data aggregated through the sensors and the app. Since the data came in various formats from multiple sources, our app automated its collection, storage, and validation.
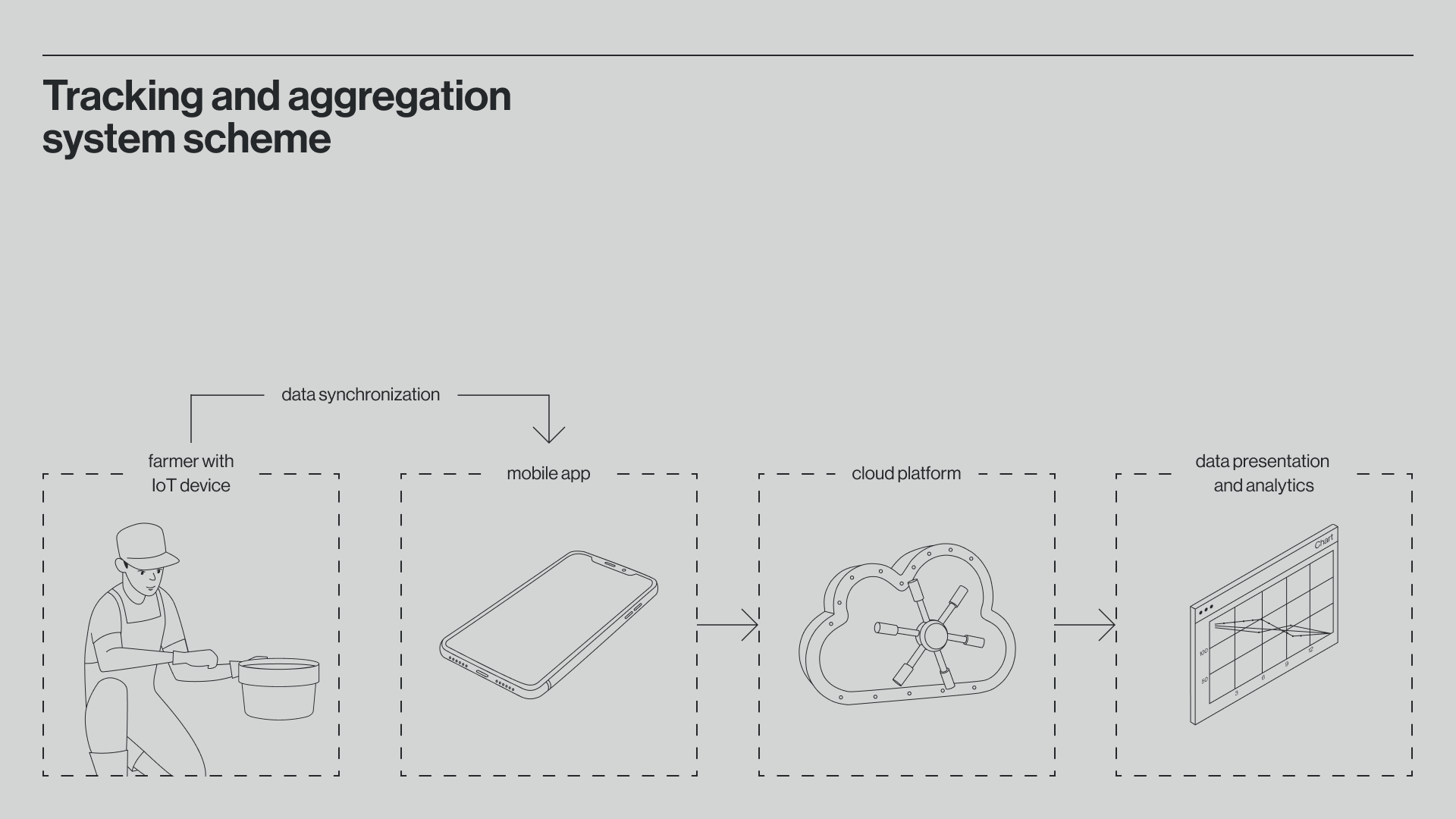
Blockchain and big data
Storing big data with traditional cloud storage providers may cost a fortune, so businesses often consider blockchain. This decentralized data storage approach solves two problems with data management:
- Security: Data on the ledger cannot be forged, so the companies know it’s secure
- Cost: It costs less to store it using blockchain than a traditional cloud-based data lake
Using blockchain and big data together improves data security, gives more control of data sharing, creates a safe environment for data monetization, and prevents fraud. What’s more, it improves verification processes, as it’s possible to track data origin.
For example, the News Provenance Project by NYT and IBM Garage aims to combat Internet misinformation by using blockchain and big data. The project helps track the source of the image and the associated data (who took it and where it was taken and posted) to detect whether this visual is credible and can accompany the news.
Big data is an integral part of modern technologies. Let’s review the major trends already shaping the future of big data.
Trends in Big Data
According to the Gartner research, there are three categories of trends in big data and analytics:
- Those activating dynamism and diversity
- Those augmenting people and decisions
- Those institutionalizing truth
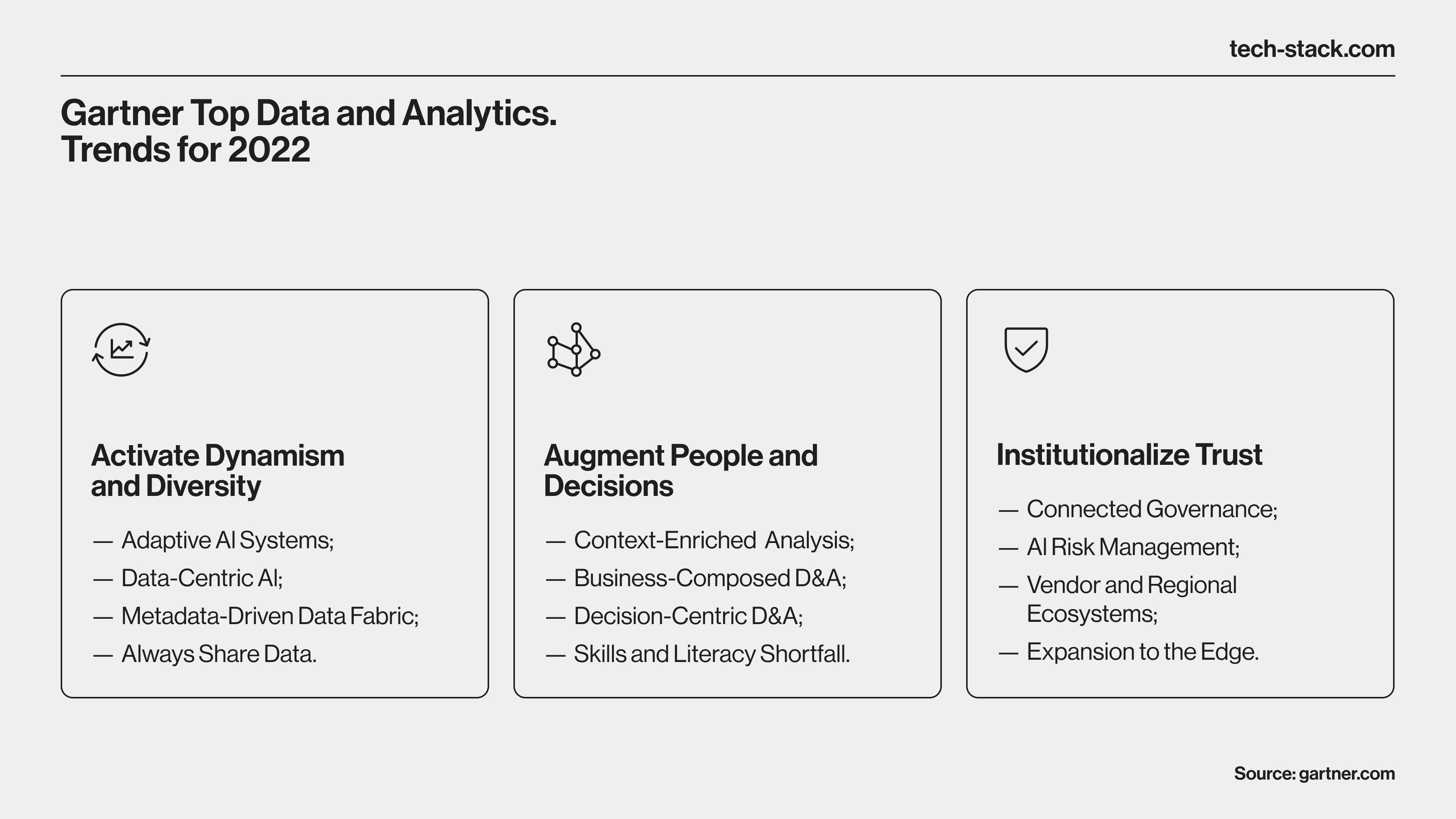
Let’s review the ones you should prioritize when addressing big data changes for your business.
Wider adoption of DataOps and data stewardship
The volume of data is growing, which makes the implementation of DataOps strategically critical for a company that wants to use it. DataOps is an Agile approach to designing, implementing, and maintaining a distributed data architecture.
Inspired by DevOps, DataOps has a few core tasks:
- Support a wide range of open-source tools and frameworks in production
- Speed up the production of apps that run on big data processing frameworks
- Remove silos across IT operations regarding data management and provide conditions for the in-house and remote software development teams, data engineers, scientists, and analysts to use available data where its use complies with regulations.
The ultimate goal of DataOps is to drive business value from the data the company receives and produces. This translates into better customer management, thorough fraud detection algorithms, and in-depth business analytics.
Another trend closely connected with DataOps is data stewardship. Contrary to big data governance, which deals with high-level policies, the data stewardship management approach focuses on tactical coordination and implementation of data. As data policies and regulations become more stringent, adopting this approach will help companies use the data for corporate and business goals risk-free.
Data-centric AI
To deliver the expected results, an AI solution should follow the data-centric approach.
Unlike model-centered AI, which focuses more on code, data-centered AI focuses on data. This data-first approach has a few benefits:
- It decreases the trial-and-error time to improve the model, reducing development time
- It promotes collaboration between developers, data scientists, and data engineers
- It improves accuracy and yields as it uses quality data sets
By creating data-centric AI, you will reduce bias in the data, improve data diversity, and streamline labeling processes. This will result in more accurate big data management required in diverse and distributed environments.
Meta-driven data fabric
The concept of data fabric–a set of data services running in the cloud environment–drives superior big data management.
Here are the processes that data fabric includes:
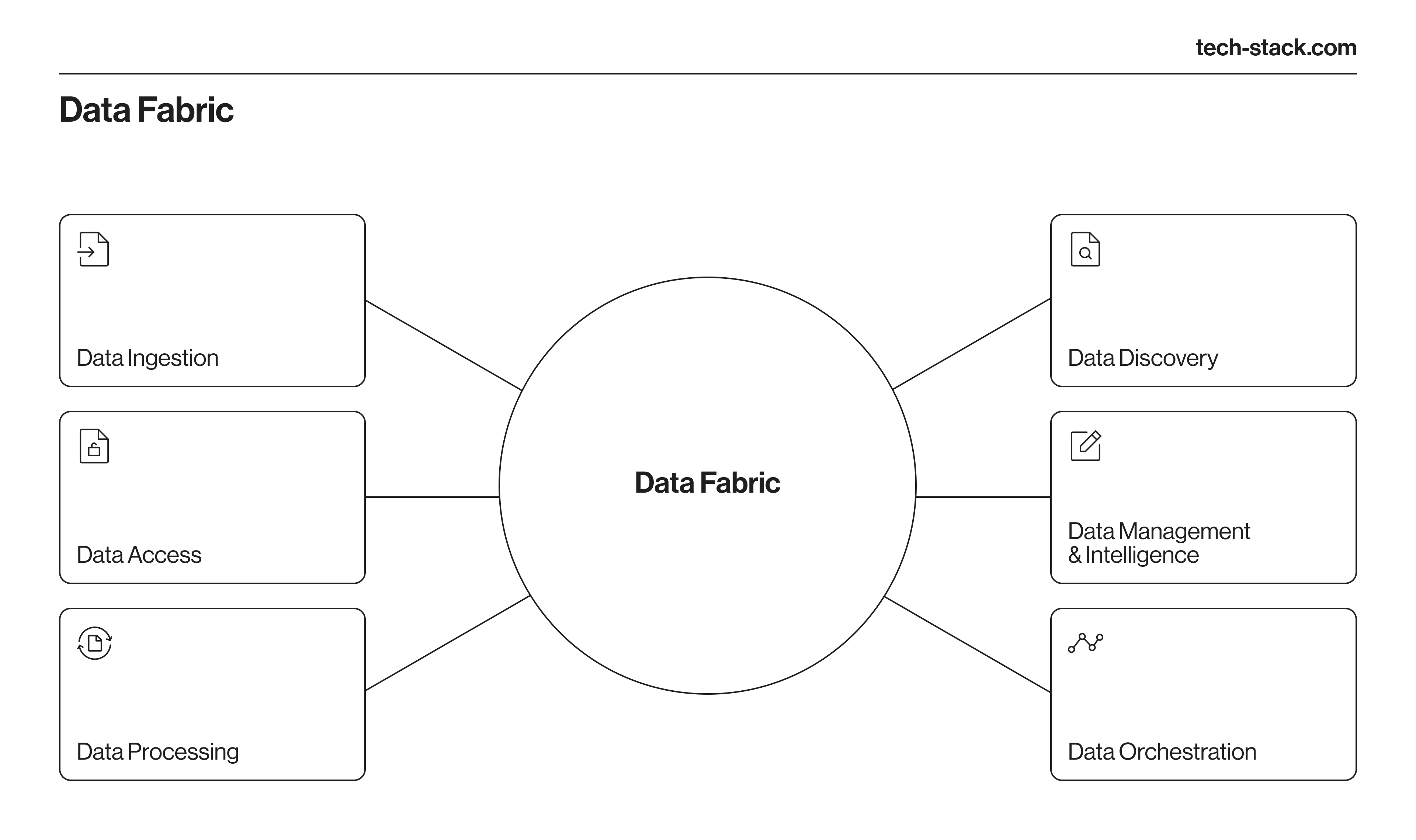
Data fabric uses metadata to flag and recommend the most efficient actions for machines and people in specific circumstances. This data architecture concept is crucial for automation.
Organizations are expected to move up to 65% of their IT services to the cloud and engage more in DevOps and microservices, so data will be managed differently after the migration. From this perspective, using a meta-driven data fabric is critical to prevent chaos and increase data visibility.
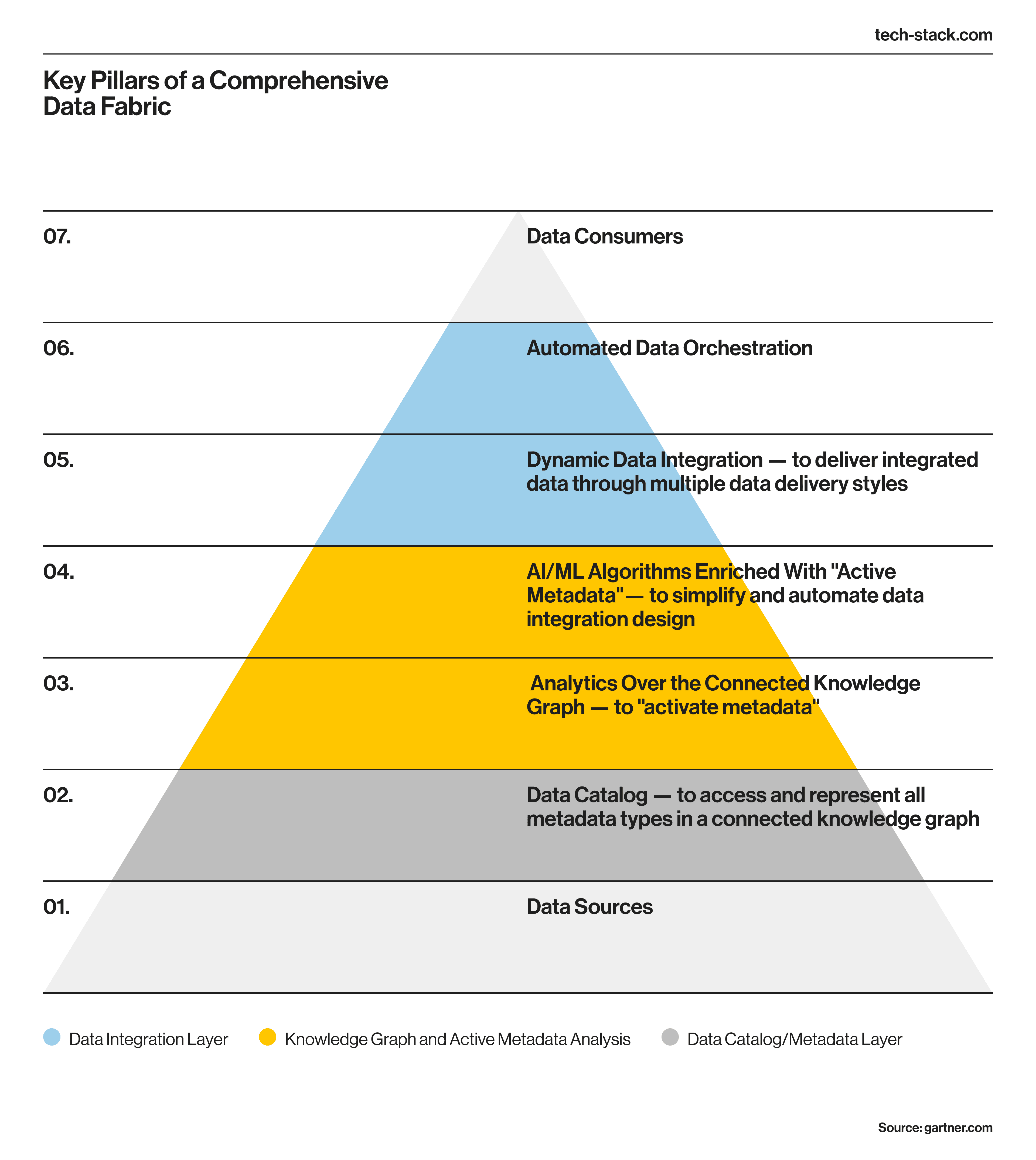
More investment in secure data sharing
Isolated data, however useful, is more of a burden than an asset. This is why companies will invest in secure data sharing.
It will often involve data architecture redesign, which will help remove silos between departments and enable safe data sharing at scale. The redesign is urgent if you partner with third-party software developers that use this data to do their job.
Creation of regional and vendor data ecosystems
One of the reasons that will force businesses to reconsider and redesign their big data sourcing and processing is the regional data regulation policies. To comply with laws (GDPR, HIPAA, CCPA, or others), global organizations must locate some or all of their data and analytics centers within particular regions.
This will cause a few critical shifts throughout companies:
- You will need to design multi-cloud or hybrid cloud management to evaluate how you will process the big data efficiently and what data can be used by specific teams
- You’ll need to create a multi-vendor strategy to ensure silo-free access to data
These changes will also alter your cooperation with third-party tech vendors, as you will need someone qualified to access, process, and store specific data.
Predictive analytics
Predictive analytics accumulates and analyses historical and real-time data to find meaningful patterns and predict future results. Big data makes these predictions more accurate.
For example, a Canadian AI platform Blue Dot detected the outbreak of COVID-19 before the World Health Organization did.
They used an AI-based algorithm that processed information like statements from official public health organizations, airline ticket data, livestock health reports, and digital media every 15 minutes daily.
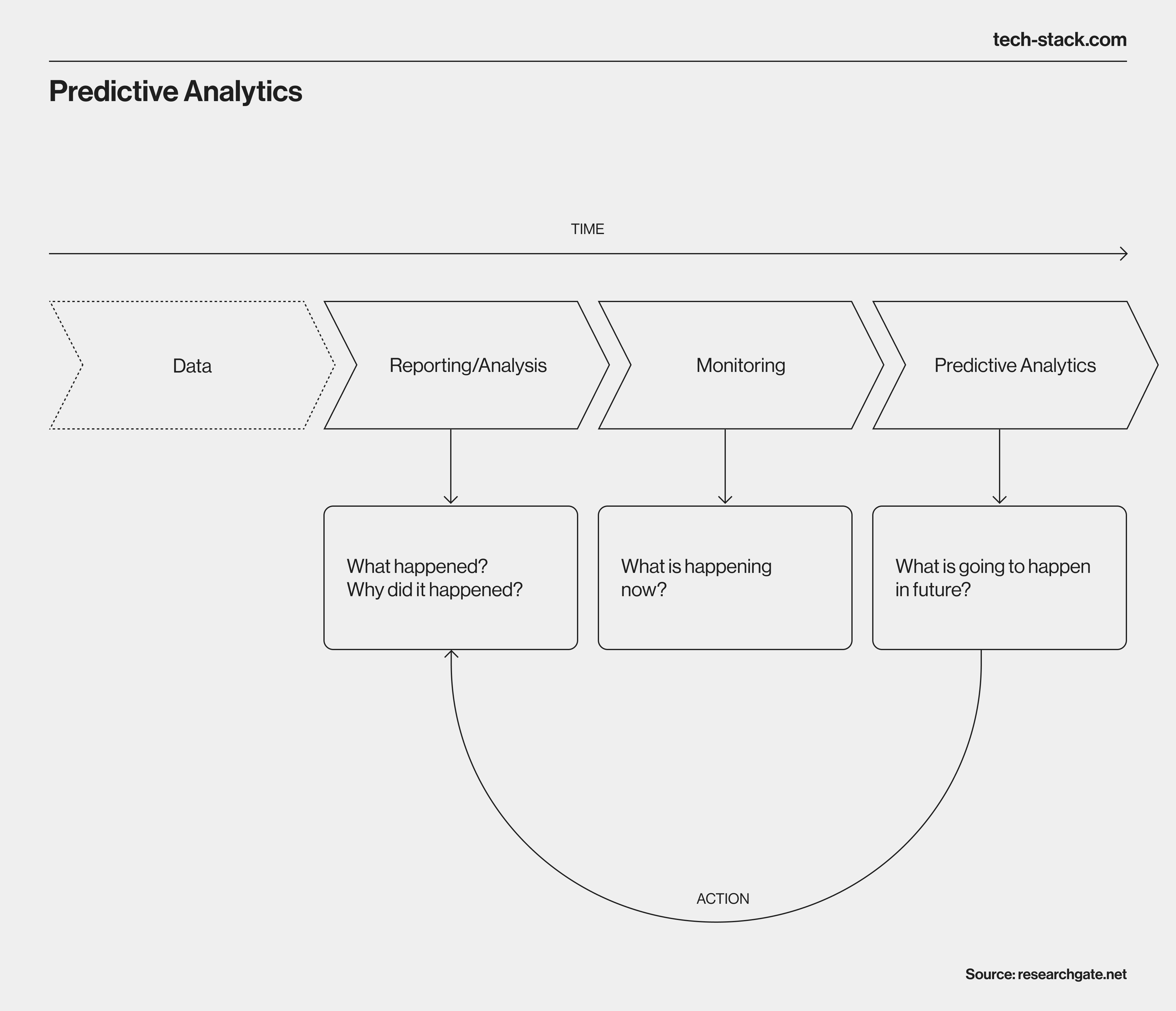
These trends show that big data is already widely used across industries. Let’s explore what the future holds in store.
The Future of Big Data
Based on the current trends and use cases, here are our predictions for the future of big data.
- Companies will continue to rely on data manipulation to analyze, predict, and swiftly adapt to changing market conditions.
- Big data analytics will guide R&D departments in creating new goods and services and proactive product development.
- Cleaned, prepared, and ready-to-share data will become a product in more markets.
- Companies will create more diverse data lakes that enable data reuse.
- More low or no-code solutions will empower non-data analytics to use ready data sets for software development and analytics. It will help establish data-driven workflows throughout the company.
Even if you don’t plan to use big data solutions now, you must start planning the data architecture redesign for the future. It is crucial if you consider implementing data-heavy AI or IoT-based solutions, as access to quality data from various sources will propel you toward the expected results.
Summing Up
Big data provides businesses with a massive amount of information from multiple channels they can structure and use. Paired with innovative technologies like IoT, blockchain, and AI, big data allows industries to generate insights, detect patterns, and optimize decision-making.
Industries like software development, fintech, healthcare, and marketing are already reaping the benefits of deploying big data. They also set the trends for the future.
Techstack provides plenty of big data and analytics services to leverage the available information. If you’re ready to unlock the power of big data for your organization, contact our team to discuss how we can help.