Imagine a system that enhances your energy grid’s reliability, reduces operating costs, and supports your sustainability goals, all while offering backup power for outages. This is essentially the promise of microgrids—independently operated small-scale grids.
Microgrids work with multiple distributed energy sources, such as solar panels, fuel cells, and wind turbines. Where outdated management systems lack the scalability and capacity to integrate essential renewable energy sources, AI works to enhance your microgrid optimization and management.
- AI systems with advanced data analytics and prediction capabilities improve decision-making, making microgrids more responsive to fluctuating production scenarios.
- Automation can optimize energy distribution on the fly.
- Thanks to ML technologies, your energy and usage predictions become more accurate over time.
In this article, we’ll dive deeper into the advantages of AI for microgrid management, using real-world cases for illustration. We’ll also tell you how to deal with the challenges of implementing AI in your networks. Finally, we’ll discuss trends in the microgrid sector to prepare you for the future.
The Role of AI in Microgrid Management
Traditional microgrid management systems struggle to process the huge volume of data generated by grid components. Legacy systems also struggle to integrate renewable energy sources into microgrids. As a result, microgrids can fail to respond to demand effectively, especially during peak periods. These problems compound as a grid incorporates more distributed energy sources.
This complexity is driving the demand for smarter microgrid controller systems for managing distributed energy sources.
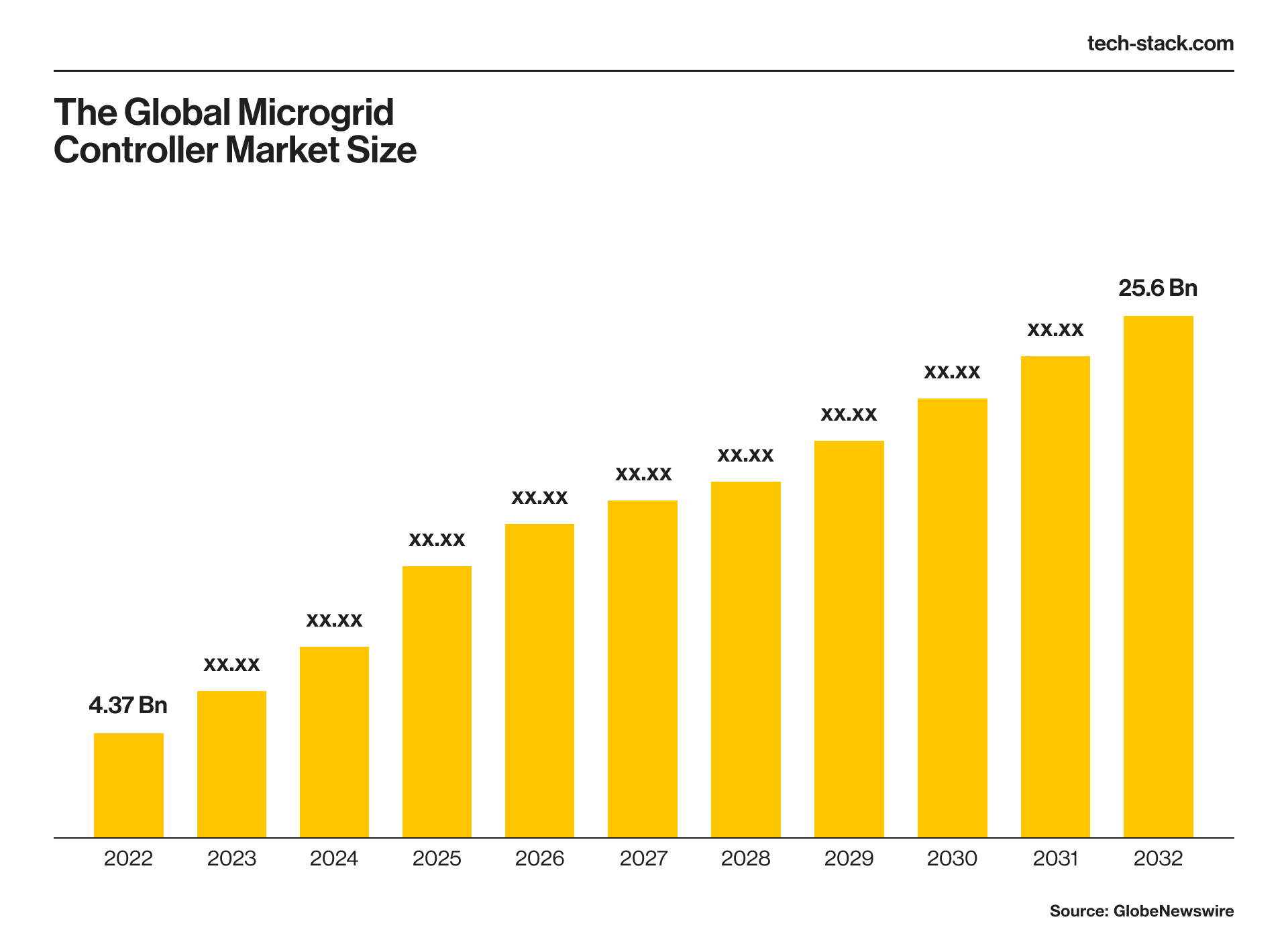
Smart, AI-based controllers solve microgrid management problems, as they can process vast datasets and make automated decisions based on them.
Let’s see what AI can bring to your microgrid optimization.
- Real-time data analysis. AI algorithms process input from a network of IoT sensors placed throughout the microgrid. Voltage levels, current flows, power outputs, light intensity, weather, and emissions are all analyzed in real time and stored for further analysis.
- Demand response management. AI systems can forecast peak demand periods based on real-time and historical data about power generation and consumption, coupled with environmental information. This lets you proactively adjust microgrid resource allocation.
- Load balancing. Advanced algorithms distribute power from energy storage systems most efficiently based on variations in supply and demand. This helps ensure peak efficiency, low chance of overload, and minimal energy losses—all critical advantages for microgrids with unpredictable renewable energy production equipment.
- Predictive maintenance. Trained ML models can identify subtle changes in a system’s performance, physical condition, or sound to anticipate microgrid maintenance needs. Upon detecting an anomaly, AI will alert personnel and schedule time for maintenance.
- Cyber threat and anomaly detection. Advanced security systems use AI to deal with cyber threats. They use pattern recognition and anomaly detection to identify potential security breaches, helping prevent costly disruptions, data theft, and safety hazards.
- Regulatory compliance improvements. Microgrid optimization software helps your company comply with complex energy regulations and environmental safety laws. You also gain an in-depth understanding of efficiency benchmarks and emissions, making it easier to meet sustainability goals.
These are all core features and advantages of AI-driven microgrid management. However, there are more sophisticated uses of AI for microgrids, too.
Advanced Use of AI for Microgrid Operations
AI technologies can essentially turn your grid into a smart system with enhanced reliability and efficiency. This is done through demand forecasting and renewable energy management.
Predictive analytics for demand forecasting
AI systems can create accurate forecasts by analyzing large amounts of data across microgrids. Meanwhile, ML algorithms learn from patterns to refine the accuracy of predictions as they receive more data.
So, what improvements can you expect after implementing advanced AI for your microgrids?
Demand prediction accuracy
AI systems with time-series analysis scrutinize energy consumption data to identify patterns and peak demand periods.
Load prediction accuracy
Detailed analytics of IoT devices allow microgrid controllers to adjust power generation and storage to maximize efficiency.
Price forecasting for trading
AI integrates market price data to predict the best time to sell energy back to the main grid and when to purchase additional power.
Consumption pattern analysis
Data mining and classification technologies help classify energy consumers based on demographics, devices used, and other factors to tailor microgrid energy distribution strategies.
Anomaly detection
Clustering and classification algorithms can be trained to recognize anomalies, such as unauthorized access attempts, equipment failure, or sudden inefficiency.
Weather impact analysis
Predictive weather modeling can forecast how weather changes will affect energy demand among consumers or in microgrids reliant on climate-sensitive renewables.
Customized pricing
AI can dynamically adjust energy prices to incentivize off-peak usage and discourage consumption during peak times.
In short, integrating advanced AI systems can enhance the efficiency of microgrid operations and reduce operational costs at all levels. What’s more, modern technologies make power generation more sustainable.
AI in renewable energy integration
Managing green energy is challenging due to its unpredictable sources. Luckily, AI software engineered for renewable energy can monitor performance metrics and external factors such as the weather. Intelligent platforms can anticipate changes and maintain a balanced energy supply by optimizing microgrid operations in different ways.
- Integration efficiency. AI coordinates inputs from all energy sources in the microgrid. It allows both renewable and traditional sources to work together for optimal energy production, storage, and distribution.
- Energy storage optimization. The system uses energy storage systems such as batteries to store excess energy generated during peak production. Algorithms then calculate the best energy release times to prevent outages and overloads.
- Intermittency management. Platforms can analyze the past performance of renewable sources from sensors and data from weather stations to optimize equipment operations. For example, if AI detects incoming cloudy weather that could reduce solar energy production, it redirects energy from other sources to compensate.
- Optimal resource allocation. Optimization algorithms calculate the most efficient distribution of energy sources based on projected demand. This helps maintain consistent voltage across the microgrid, ensuring a reliable power supply.
- Environmental impact monitoring. AI systems monitor the environmental impact of energy sources within the microgrid, including calculating emissions. What’s more, AI-powered systems can configure your microgrid to prioritize greener sources whenever possible, switching to traditional methods only when necessary to avoid outages.
Custom software is a great choice for making renewable energy more accessible. However, without prior experience, this technology is difficult to integrate into a legacy IT environment.
Challenges and Solutions in AI-Driven Microgrid Optimization
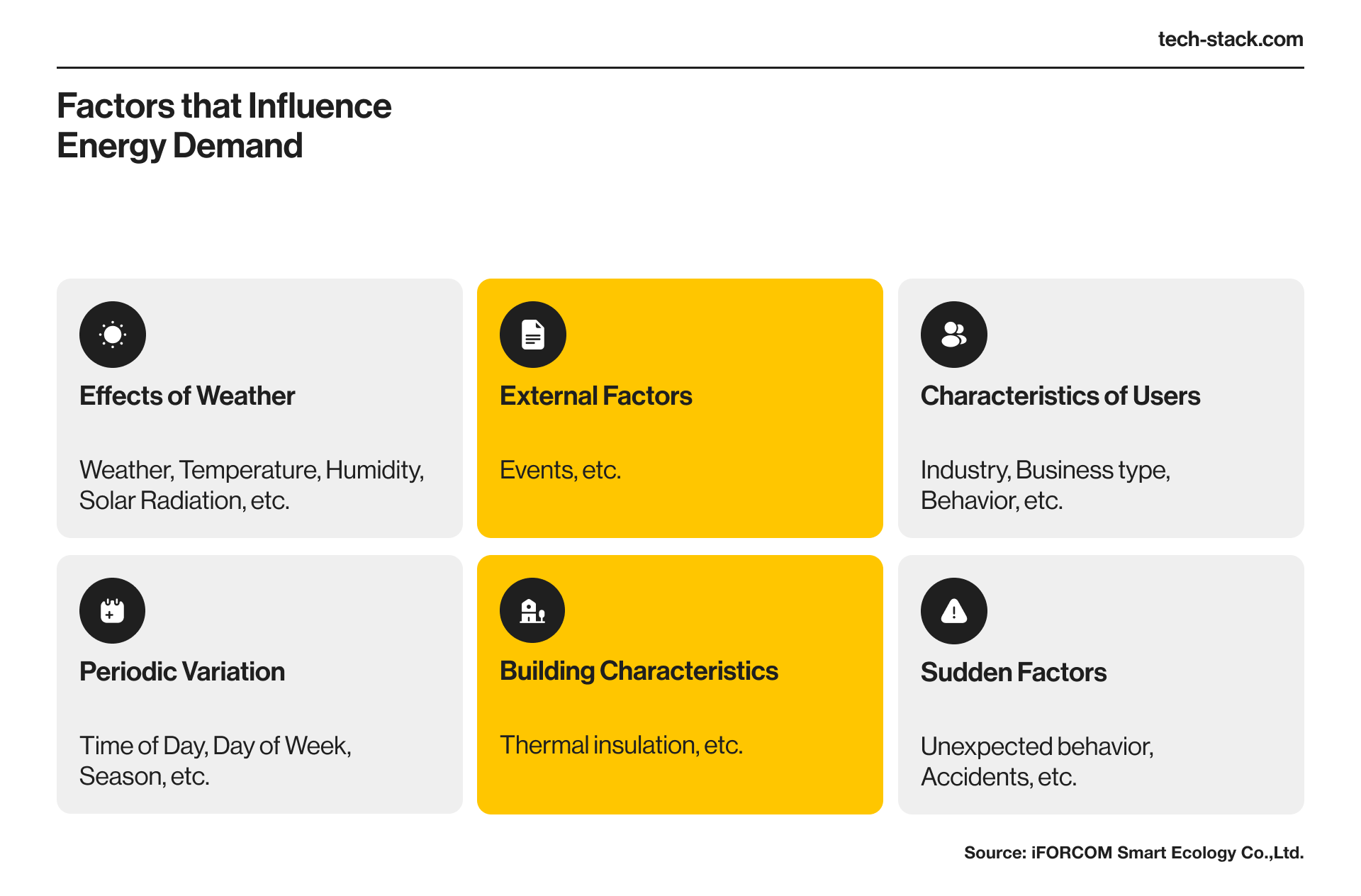
AI, ML, and big data analytics offer huge promise, but existing systems must be optimized to reap their benefits. This requires an understanding of the challenges and how to address them.
Compatibility with existing infrastructure
Microgrids often rely on outdated systems that lack connectivity with modern data standards and cloud infrastructures. Yet legacy systems and IoT networks must communicate with sophisticated AI applications for real-time monitoring and predictive analysis.
Solutions:
- Plan microgrid modernization in phases
- Start by optimizing non-critical applications and operations to minimize disruption to existing workflows
- Build custom middleware and APIs to facilitate data exchange between legacy technologies and AI software
Energy storage optimization
Poorly designed algorithms may manage networks inefficiently or fail to discharge energy storage systems at peak times. Badly managed batteries can leak excess energy or lead to premature battery degradation, which leads to excess costs.
Solutions:
- Choose reliable AI software that accurately determines storage discharge times based on historical data and real-time inputs
- Train ML models on verified datasets to improve prediction accuracy
Training data quality
The accuracy of your predictions depends on the quality and completeness of the data. Without this, AI systems can misinterpret energy needs, leading to misguided load distribution and demand forecasting.
Solutions:
- Set up comprehensive data collection and processing tools for IoT sensor data
- Implement data governance frameworks to verify datasets for discrepancies, corrupted files, and other errors
- Integrate data from multiple geographic locations, consumer profiles, and timeframes to avoid bias in AI predictions
Security and privacy risks
Integrating IoT devices in a microgrid increases the number of potential access points for hackers. Meanwhile, personally identifiable information needs to be stored and used in accordance with regulations like GDPR and the CCPA.
Solutions:
- Choose a ready-made or custom AI solution with high-level encryption and authentication methods to secure data transmission and minimize the risk of unauthorized access
- Implement AI-enhanced threat detection and prevention systems to respond to suspicious network activity and mitigate breaches
- Conduct regular vulnerability assessments and privacy audits to identify weaknesses in IoT infrastructure and associated software
- Use synthetic data generation tools to create representative training data without risking privacy infringement
System scalability
Your AI management platform needs to cope with an increase in the number of devices in your microgrid network. Poor scalability will lead to system overload and slow down transactions and decision-making.
Solutions:
- Migrate to a cloud-based or hybrid infrastructure to manage peaks in data volume and processing demand without costly physical upgrades
- Opt for a platform with auto-scaling support for a more agile response to system demand
- Adopt edge computing to optimize latency by placing smaller data processing units near clusters of IoT devices
A wide skills gap
AI-powered optimization technologies for microgrids require specific expertise. The same skills gap exists for green computation and climate change expertise.
LinkedIn’s 2023 Global Green Skills Report suggests that only one in eight workers has at least one green skill—far below what the current economy needs, given a year-on-year increase of just over 15% in the hiring rate for workers with these skills in 2023.
Solutions:
- Collaborate with expert training providers to develop customized training modules for your workforce
- Check for built-in training modules and extensive guides to make it easier for employees to learn your platform’s features
- Set up a continuous professional development program to keep employee skills aligned with developments in green energy
Advanced AI systems in microgrids demand significant upfront and long-term investment. While this is challenging, practice shows that a reliable software development company can make implementation easier.
Case Studies: AI in Action for Microgrid Optimization
Case studies prove the benefits of AI for microgrid energy management. Let’s look at some examples.
Solar energy storage system
When an energy company based in Los Angeles, USA, faced challenges integrating a custom energy storage system into their complex EMS, they turned to Techstack for a solution. The project required a system with solar panels, batteries, and photovoltaic inverters.
Research showed us that existing open-source platforms were insufficient for the project requirements. In response, we developed a custom software solution for solar energy storage. The system we developed is now deployed in a testing environment, and we are enhancing the existing functionality.
Results:
- Our solution facilitates integrating renewable energy systems into smart grid architectures for optimal solar energy utilization.
- Our partner has significantly reduced its carbon footprint thanks to AI-powered energy resource management.
- Using advanced software to optimize energy usage and incorporate predictive maintenance has led to valuable savings in operating costs.
You can learn more about the project here.
Energy balancing platform
A Finnish energy company wanted to build an energy balancing system optimized for off-peak energy periods. We built a piece of custom software that tracks and streamlines bids, saves data into a database, and tracks energy consumption.
Results:
- Our software efficiently tracks and stores transactions and energy usage data.
- The company and its clients can use the intuitive report and invoice-generation tools.
- The platform includes basic features for managing and tracking energy consumption and bills.
- We developed an application dedicated to managing hardware connections and system load that can be used for microgrid optimization.
You can learn more about the project here.
Microgrid data center assistance system
US energy provider Vertiv created a microgrid power solution for data center management. The system allows companies to evaluate how well a microgrid can work with existing data center infrastructure technologies.
Results:
- The platform analyzes the microgrid's reliability, backup duration, and responsiveness under normal and extreme conditions, helping ensure reliable operations at peak times.
- The integrations with distributed energy resources and battery energy storage systems reduce the risks associated with utility outages.
- This system also acts as a performance test lab, enabling companies to optimize microgrid configurations for specific data center requirements.
Read more about the project and results here.
Solar energy data portal
We created a solar energy production platform for a partner in New York. The solution brings the complex and heterogeneous data formats, standards, and communication protocols used by different providers into a single platform and ensures the interoperability of diverse network components.
Results:
- We developed a complex platform that collects solar energy data hourly, including historical data.
- Users can navigate the portal to access real-time and historical data analyses.
- The platform's architecture allows for easy integration of new services, IoT devices, and features.
You can learn more about the project here.
These results are exciting, but they’re just a start when you consider the advances in big data analytics, AI, and ML.
The Future of AI in Microgrid Optimization
Current uses and trends point to a bright future for AI technology. Here’s what we can expect to see in the coming years.
- The rise of AI-based energy storage systems for microgrids. Industry forecasts suggest that the global energy storage market will grow from $198.8 billion in 2022 to $329.1 billion in 2032. Intermittent energy sources require extra storage systems to maintain stability in microgrids.
- Reliance on distributed energy resource systems. Businesses continue investing in distributed energy generation systems and AI software as an alternative to long-distance transmission lines. The global distributed energy market is predicted to reach $916.16 billion by 2032, nearly 3.5 times its value in 2022.
- Virtual power plants for microgrids. VPPs are AI-driven platforms that manage grid-based power-generating units and energy consumers. They can optimize microgrid stability by acting as a bridge between renewable energy sources and traditional power markets. The VPP market is expected to see a 22% CAGR between 2023 and 2032.
- Advanced remote asset monitoring tools. Traditional monitoring methods are still impractical for hardware in remote locations. As a result, we’ll likely see the development of more advanced sensors, AI-enhanced cameras, and autonomous drones to monitor performance, emissions, and hard-to-reach places.
- Cost-effective MaaS solutions. Microgrid-as-a-Service (MaaS) companies take on the deployment of microgrid control systems, allowing businesses to pay only for utilized energy. This option requires little upfront investment and reduces most financial risks. The global MaaS market is set to grow from $1.85 billion in 2021 to $7.83 billion by 2031.
Microgrid AI systems are central to many of these developments as the need for decentralization and renewable energy increases.
Turn to AI for Effective Microgrid Management
A reliable AI system covers what happens behind the scenes in your microgrid network. Smart algorithms and AI can optimize microgrid operations based on massive volumes of data and generate predictions to keep your microgrid running competitively.
Using a scalable AI-powered platform for microgrid optimization can even increase your energy production capacity. And, if existing solutions don’t cover your needs, you can partner with a reliable vendor like Techstack for a custom AI development solution. With ten years of development experience and a portfolio of ambitious energy projects, we’d love to create your energy solution.
Want to upgrade your microgrid to a data-driven smart grid? Reach out to our team to learn about the possibilities.